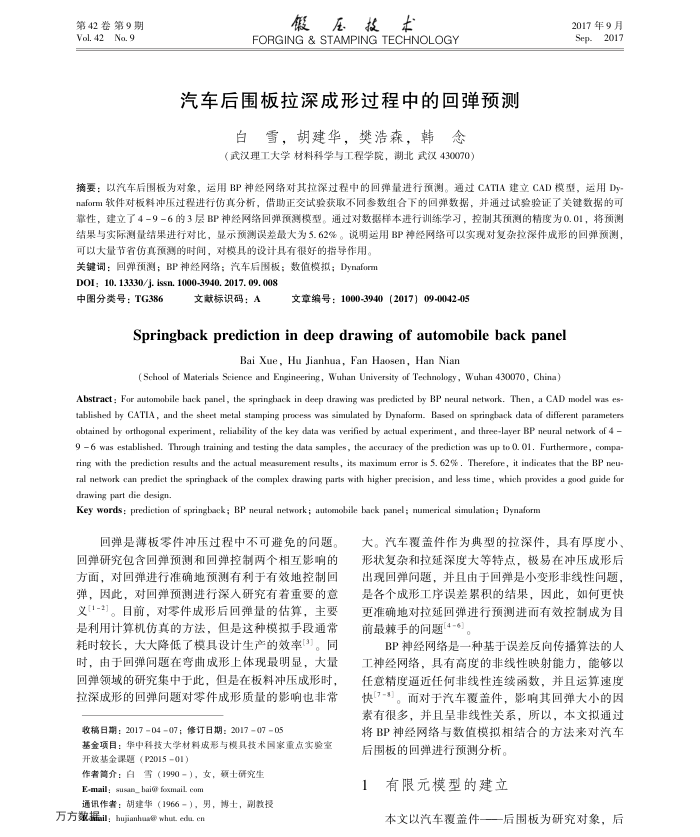
第42卷第9期 Vol. 42No.9
假压技木
FORGING & STAMPING TECHNOLOGY
汽车后围板拉深成形过程中的回弹预测
白雪,胡建华,樊浩森,韩念(武汉理工大学材料科学与工程学院,湖北武汉430070)
2017年9月 Sep.2017
摘要:以汽车后围板为对象,运用BP神经网络对其拉深过程中的回弹量进行预测。通过CATIA建立CAD模型,运用Dy nafom软件对板料冲压过程进行仿真分析,借助正交试验获取不同参数组合下的回弹数据,并通过试验验证了关键数据的可靠性,建立了4-9-6的3层BP神经网络回弹预测模型。通过对数据样本进行训练学习,控制其预测的精度为0.01,将预测结果与实际测量结果进行对比,显示预测误差最大为5.62%。说明运用BP神经网络可以实现对复杂拉深件成形的回弹预测,
可以大量节省仿真预测的时间,对模具的设计具有很好的指导作用关键词:回弹预测;BP神经网络;汽车后围板;数值模拟;Dynaform DOI: 10. 13330/j. issn. 1000-3940. 2017. 09, 008
中图分类号:TG386
文献标识码:A
文章编号:1000-3940(2017)09-0042-05
Springback prediction in deep drawing of automobile back panel
Bai Xue, Hu Jianhua, Fan Haosen, Han Nian
( School of Materials Science and Engineering, Wuhan University of Technology, Wuhan 430070, China)
Abstract : For automobile back panel, the springhack in deep drawing was predicted by BP neural network. Then, a CAD model was es tablished by CATIA, and the sheet metal stamping process was simulated by Dynafom. Based on springhack data of different parameters obtained by orthogonal experiment, reliability of the key data was verified by actual experiment, and three-layer BP neural network of 4 9 6 was established. Through training and testing the data samples, the accuracy of the prediction was up to O. 01. Furthemore, compa-ring with the prediction results and the actual measurement results, its maximum ernor is 5. 62% . Therefore, it indicates that the BP neu-ral network can predict the springhack of the complex drawing parts with higher precision, and less time, which provides a good guide for drawing part die design.
Key words : prediction of springback; BP neural network ; automobile back panel; numerical simulation; Dynaform
回弹是薄板零件冲压过程中不可避免的问题。回弹研究包含回弹预测和回弹控制两个相互影响的方面,对回弹进行准确地预测有利于有效地控制回弹,因此,对回弹预测进行深人研究有着重要的意义(1-2]。目前,对零件成形后回弹量的估算,主要是利用计算机仿真的方法,但是这种模拟手段通常耗时较长,大大降低了模具设计生产的效率[3]。
间
时,由于回弹问题在弯曲成形上体现最明显,大量回弹领域的研究集中于此,但是在板料冲压成形时,拉深成形的回弹问题对零件成形质量的影响也非常
收稿日期:20170407;修订日期:20170705
基金项目:华中科技大学材料成形与模具技术国家重点实验室开效基金课题(P2015-01)
雪(1990),女,额土研究生
作者简介:白
E-mail: susan_hai@ foxmail. com
通讯作者:胡建华(1966-),男,博士,副教投万方数据ail:hujianhua@ whut eda. cn
大。汽车覆盖件作为典型的拉深件,具有厚度小、形状复杂和拉延深度大等特点,极易在冲压成形后出现回弹问题,并且由于回弹是小变形非线性问题,是各个成形工序误差累积的结果,因此,如何更快更准确地对拉延回弹进行预测进而有效控制成为目前最棘手的间题[4-6]。
BP神经网络是一种基于误差反向传播算法的人工神经网络,具有高度的非线性映射能力,能够以任意精度逼近任何非线性连续函数,并且运算速度快[7-3]。面对于汽车覆盖件,影响其回弹大小的因素有很多,并且呈非线性关系,所以,本文拟通过将BP神经网络与数值模拟相结合的方法来对汽车后围板的回弹进行预测分析。
1
有限元模型的建立
本文以汽车覆盖件—后围板为研究对象,后