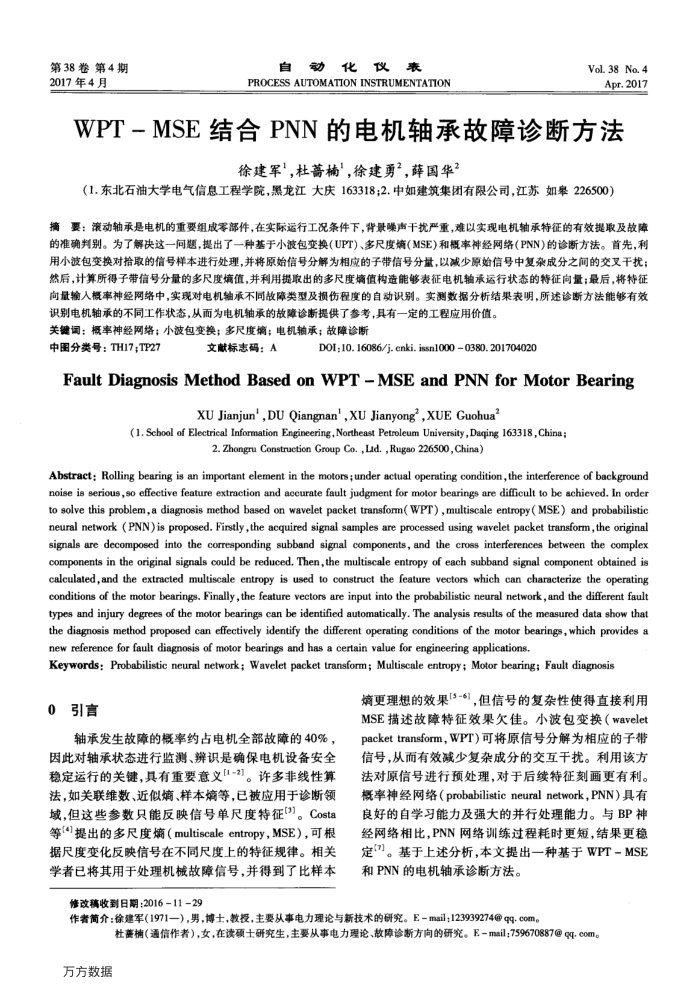
第38卷第4期 2017年4月
自
动化
仪
表
PROCESS AUTOMATION INSTRUMENTATION
Vol. 38 No. 4 Apr.2017
WPT-MSE结合PNN的电机轴承故障诊断方法
徐建军",杜蔷楠",徐建勇’,薛国华
(1.东北石油大学电气信息工程学院,黑龙江大庆163318;2.中如建筑集团有限公司,江苏如阜226500)
摘要:滚动轴承是电机的重要组成零部件,在实际运行工况条件下,背景噪声干扰严重,难以实现电机轴承特征的有效提取及故障的准确判别。为了解决这一问题,提出了一种基于小波包变换(UPT)、多尺度缩(MSE)和概率神经网络(PNN)的诊断方法。首先,利用小波包变换对拾取的信号样本进行处理,并将原始信号分解为相应的子带信号分量,以减少原始信号中复杂成分之间的交叉干扰然后,计算所得子带信号分量的多尺度摘值,并利用提取出的多尺度摘值构造能够表征电机轴承运行状态的特征向量;最后,将特征向量输人概率神经网络中,实现对电机轴承不同故障类型及损伤程度的自动识别。实测数据分析结果表明,所述诊断方法能够有效
识别电机轴承的不同工作状态,从而为电机轴承的故障诊断提供了参考,具有一定的工程应用价值。关键词:概率神经网络;小波包变换;多尺度摘;电机轴承;故障诊断
中图分类号:TH17;TP27
文献标患码:A
D0I:10.16086/j. enki. issn1000 0380.201704020
FaultDiagnosisMethodBasedonWPT-MSEandPNNforMotorBearing
XU Jianjun',DU Qiangnan',XU Jianyong,XUE Guohua”
(1. School of Electrical Information Engineering,Northeast Petroleum University,Daqing 163318,China ;
2. Zhongru Construetion Group Co. ,Lad. ,Rugao 226500, China)
Abstract: Rolling bearing is an important element in the motors ;under actual operating condition,the interference of background noise is serious ,so effective feature extraction and accurate fault judgment for motor bearings are difficult to be achieved. In order to solve this problem,a diagnosis method based on wavelet packet transform( WPT) ,multiscale entropy(MSE) and probabilistic neural network ( PNN)is proposed, Firstly,the acquired signal samples are processed using wavelet packet transform,the original signals are decomposed into the corresponding subband signal components, and the cross interferences between the complex components in the original signals could be reduced. Then,the multiscale entropy of each subband signal component obtained is calculated,and the extracted multiscale entropy is used to construct the feature vectors which can characterize the operating conditions of the motor bearings. Finally ,the feature vectors are input into the probabilistic neural network, and the different fault types and injury degrees of the motor bearings can be identified automatically, The analysis results of the measured data show that the diagnosis method proposed can effectively identify the different operating conditions of the motor bearings, which provides a new reference for fault diagnosis of motor bearings and has a certain value for engineering applications.
Keywords: Probabilistic neural network; Wavelet packet transform; Multiscale entropy; Motor bearing; Fault diagnosis
0
引言
轴承发生故障的概率约占电机全部故障的40%,
因此对轴承状态进行监测、辨识是确保电机设备安全稳定运行的关键,具有重要意义[1-2]。许多非线性算法,如关联维数、近似熵、样本摘等,已被应用于诊断领域,但这些参数只能反映信号单尺度特征[3]。Costa 等(4提出的多尺度摘(multiscaleentropy,MSE),可根据尺度变化反映信号在不同尺度上的特征规律。相关学者已将其用于处理机械故障信号,并得到了比样本
摘更理想的效果[5-6),但信号的复杂性使得直接利用 MSE描述故障特征效果欠佳。小波包变换(wavelet packettransform,WPT)可将原信号分解为相应的子带信号,从而有效减少复杂成分的交互干扰。利用该方法对原信号进行预处理,对于后续特征刻画更有利。概率神经网络(probabilisticneuralnetwork,PNN)具有良好的自学习能力及强大的并行处理能力。与BP神经网络相比,PNN网络训练过程耗时更短,结果更稳定‘)。基于上述分析,本文提出一种基于WPT-MSE 和PNN的电机轴承诊断方法。
修改稿收到日期:2016-11-29
作者简介:徐建军(1971—),男,博士,敦授,主要从事电力理论与新技术的研究。E-mail:123939274@qq.com。
杜蓄楠(通信作者),女,在读颈士研究生,主要从事电力理论、故障诊断方向的研究。E-mail;759670887@qq.com
万方数据