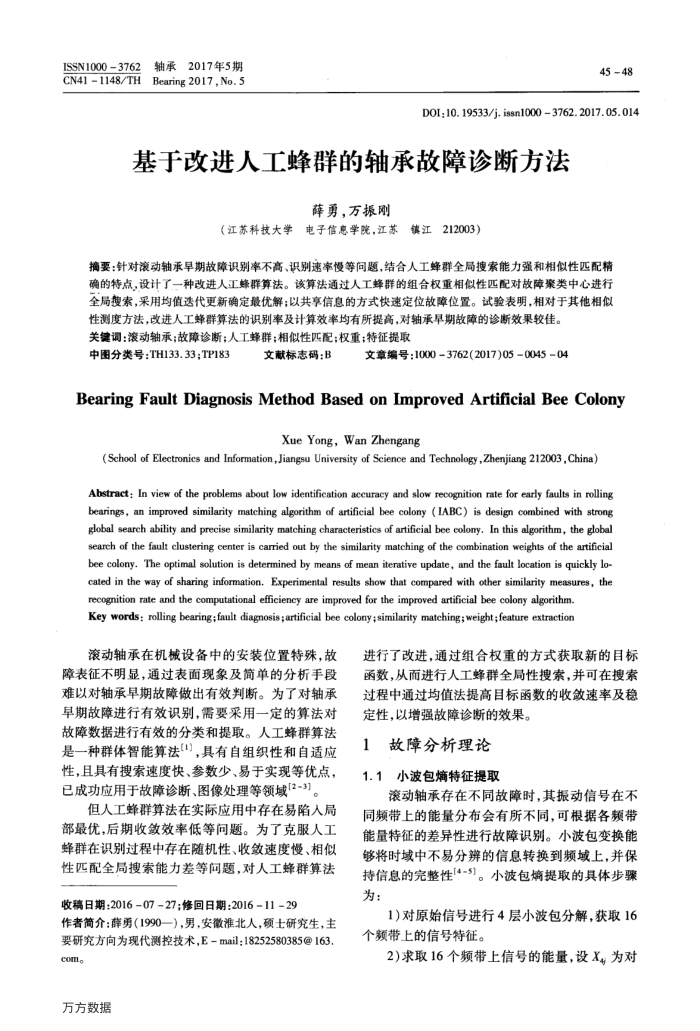
ISSN10003762 CN41 =1148/TH
轴承2017年5期 Bearing 2017, No. 5
4548
DOI; 10. 19533/j. issn1000 3762. 2017. 05. 014
基于改进人工蜂群的轴承故障诊断方法
薛勇,万振刚
(江苏科技大学电子信息学院,江苏镇江212003)
摘要:针对滚动轴承早期故障识别率不高、识别速率慢等问题,结合人工蜂群全局搜索能力强和相似性匹配精确的特点,设计了一种改进人工蜂群算法。该算法通过人工蜂群的组合权重相似性匹配对故障聚类中心进行全局搜索,采用均值选代更新确定最优解;以共享信息的方式快速定位故障位置。试验表明,相对于其他相似
性测度方法,改进人工蜂群算法的识别率及计算效率均有所提高,对轴承早期故障的诊断效果较佳。关键调:滚动轴承;故障诊断;人工蜂群;相似性匹配:权重:特征提取
中图分类号:TH133.33;TP183
文献标志码:B
文章编号:10003762(2017)05004504
Bearing Fault Diagnosis Method Based on Improved Artificial Bee Colony
XueYong,Wan Zhengang
(School of Electronics and Information,Jiangsu University of Science and Technology ,Zhenjiang 212003, China) Abstract: In view of the problems about low identification accuracy and slow recognition rate for early faults in rolling bearings, an improved similarity matching algorithm of artificial bee colony ( IABC) is design combined with strong global search ability and precise similarity matching characteristics of artificial bee colony. In this algorithm, the global search of the fault clustering center is carried out by the similarity matching of the combination weights of the artificial bee colony. The optimal solution is determined by means of mean iterative update, and the fault location is quickly lo-cated in the way of sharing information. Experimental results show that compared with other similarity measures, the
eooee rno arde aa eooa ee Key words: rolling bearing;fault diagnosis;artificial bee colony;similarity matching; weight;feature extraction
滚动轴承在机械设备中的安装位置特殊,故障表征不明显,通过表面现象及简单的分析手段难以对轴承早期故障做出有效判断。为了对轴承早期故障进行有效识别,需要采用一定的算法对故障数据进行有效的分类和提取。人工蜂群算法是一种群体智能算法"],具有自组织性和自适应性,且具有搜索速度快、参数少、易于实现等优点,已成功应用于故障诊断、图像处理等领域[2-3]
但人工蜂群算法在实际应用中存在易陷人局部最优,后期收敛效率低等问题。为了克服人工蜂群在识别过程中存在随机性、收敛速度慢、相似性匹配全局搜索能力差等问题,对人工蜂群算法
收稿日期:2016-07-27;修回日期:2016-11-29
作者简介:薛勇(1990一),男,安徽准北人,硕士研究生,主要研究方向为现代测控技术,E-mail:18252580385@163 com。
万方数据
进行了改进,通过组合权重的方式获取新的目标函数,从而进行人工蜂群全局性搜索,并可在搜索过程中通过均值法提高目标函数的收敛速率及稳定性,以增强故障诊断的效果。
故障分析理论 1
1.1小波包炳特征提取
滚动轴承存在不同故障时,其振动信号在不同频带上的能量分布会有所不同,可根据各频带能量特征的差异性进行故障识别。小波包变换能够将时域中不易分辨的信息转换到频域上,并保持信息的完整性[4-5]。小波包摘提取的具体步骤为:
1)对原始信号进行4层小波包分解,获取16 个额带上的信号特征。
2)求取16个频带上信号的能量,设X为对