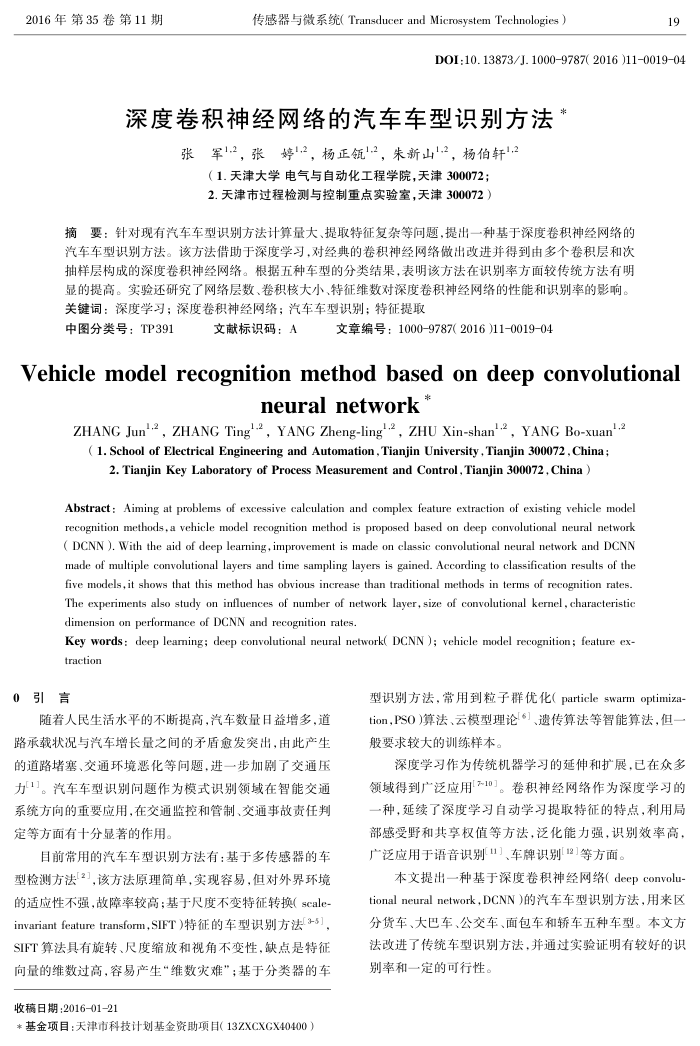
2016年第35卷第11期
传感器与微系统(Transducerand Microsystem Technologies)
19
D0I:10.13873/J.10009787(2016 )11-001904
深度卷积神经网络的汽车车型识别方法
张军"2,张婷12,杨正"2,朱新山1.2,杨伯轩1.2
(1.天津大学电气与自动化工程学院,天津300072; 2.天津市过程检测与控制重点实验室,天津300072)
摘要:针对现有汽车车型识别方法计算量大、提取特征复杂等问题,提出一种基于深度卷积神经网络的汽车车型识别方法。该方法借助于深度学习,对经典的卷积神经网络做出改进并得到由多个卷积层和次抽样层构成的深度卷积神经网络。根据五种车型的分类结果,表明该方法在识别率方面较传统方法有明显的提高。实验还研究了网络层数、卷积核大小、特征维数对深度卷积神经网络的性能和识别率的影响,关键词:深度学习;深度卷积神经网络;汽车车型识别;特征提取
中图分类号:TP391
文献标识码:A
文章编号:1000-9787(2016)11-0019-04
Vehiclemodelrecognitionmethodbasedondeepconvolutional
neuralnetwork*
ZHANG Jun-2, ZHANG Ting*2, YANG Zheng-ling-2, ZHU Xin-shan'-2, YANG Bo-xuan'.2( 1. School of Electrical Engineering and Automation, Tianjin University, Tianjin 300072, China ;
2. Tianjin Key Laboratory of Process Measurement and Control, Tianjin 300072, China)
Abstract : Aiming at problems of excessive calculation and complex feature extraction of existing vehicle model recognition methods, a vehicle model recognition method is proposed based on deep convolutional neural network( DCNN ). With the aid of deep learning, improvement is made on classic convolutional neural network and DCNN made of multiple convolutional layers and time sampling layers is gained. According to classification results of the five models,it shows that this method has obvious increase than traditional methods in terms of recognition rates. The experiments also study on influences of number of network layer, size of convolutional kernel, characteristic dimension on performance of DCNN and recognition rates
Key words : deep leaming; deep convolutional neural network( DCNN ); vehicle model recognition; feature ex: traction
0
随着人民生活水平的不断提高,汽车数量日益增多,道路承载状况与汽车增长量之间的矛盾愈发突出,由此产生的道路堵塞、交通环境恶化等间题,进一步加剧了交通压力1。汽车车型识别间题作为模式识别领域在智能交通系统方向的重要应用,在交通监控和管制、交通事故责任判定等方面有十分显著的作用。
目前常用的汽车车型识别方法有:基于多传感器的车型检测方法2],该方法原理简单,实现容易,但对外界环境的适应性不强,故障率较高;基于尺度不变特征转换(scale invariantfeaturetransform,SIFT)特征的车型识别方法[3-5], SIFT算法具有旋转、尺度缩放和视角不变性,缺点是特征向量的维数过高,容易产生“维数灾难”;基于分类器的车
收稿日期:2016-01-21
*基金项目:天津市科技计划基金资助项目(13ZXCXGX40400)
型识别方法,常用到粒子群优化(particleswarmoptimiza tion,PSO)算法、云模型理论」、遗传算法等智能算法,但一般要求较大的训练样本。
深度学习作为传统机器学习的延伸和扩展,已在众多领域得到广泛应用7-10]。卷积神经网络作为深度学习的一种,延续了深度学习自动学习提取特征的特点,利用局部感受野和共享权值等方法,泛化能力强,识别效率高广泛应用于语音识别11」、车牌识别12|等方面。
本文提出一种基于深度卷积神经网络(deep convolu tionalneuralnetwork,DCNN)的汽车车型识别方法,用来区分货车、大巴车、公交车、面包车和轿车五种车型。本文方法改进了传统车型识别方法,并通过实验证明有较好的识别率和一定的可行性。