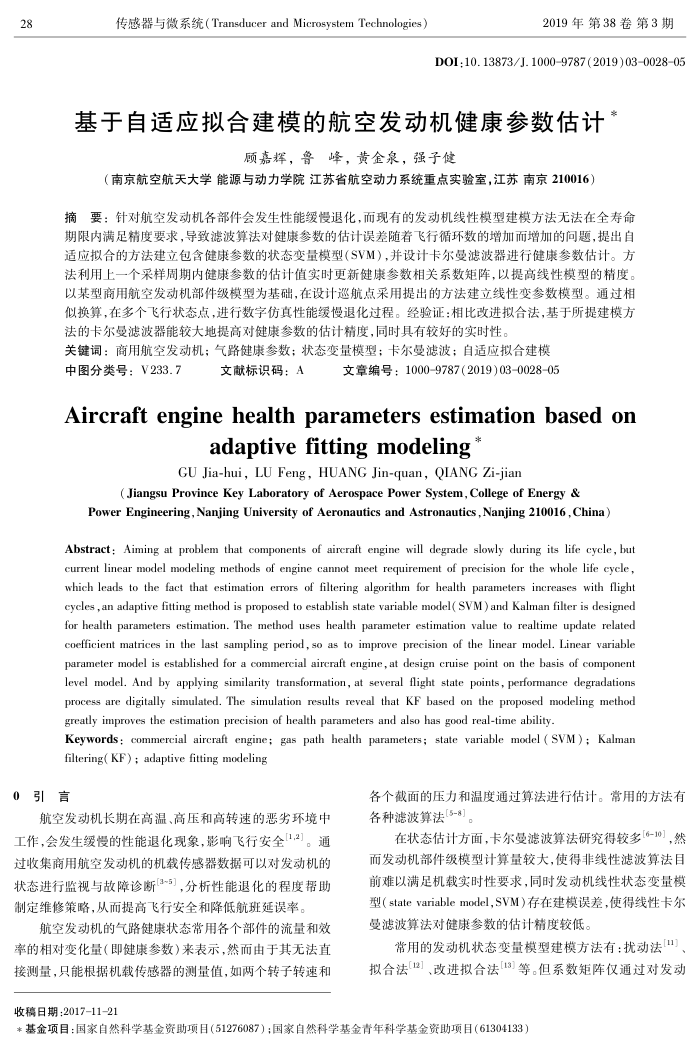
28
传感器与微系统(Transducerand MicrosystemTechnologies)
2019年第38卷第3期
DOI:10.13873/J.10009787(2019)03002805
基于自适应拟合建模的航空发动机健康参数估计
顾嘉辉,鲁峰,黄金泉,强子健
(南京航空航天大学能源与动力学院江苏省航空动力系统重点实验室,江苏南京210016
摘要:针对航空发动机各部件会发生性能缓慢退化,而现有的发动机线性模型建模方法无法在全寿命期限内满足精度要求,导致滤波算法对健康参数的估计误差随者飞行循环数的增加而增加的问题,提出自适应拟合的方法建立包含健康参数的状态变量模型(SVM),并设计卡尔曼滤波器进行健康参数估计。方法利用上一个采样周期内健康参数的估计值实时更新健康参数相关系数矩阵,以提高线性模型的精度。以某型商用航空发动机部件级模型为基础,在设计巡航点采用提出的方法建立线性变参数模型。通过相似换算,在多个飞行状态点,进行数字仿真性能缓慢退化过程。经验证:相比改进拟合法,基于所提建模方法的卡尔曼滤波器能较大地提高对健康参数的估计精度,同时具有较好的实时性。
关键词:商用航空发动机;气路健康参数;状态变量模型;卡尔曼滤波;自适应拟合建模
中图分类号:V233.7
文献标识码:A
文章编号:1000-9787(2019)03-0028-05
Aircraft enginehealth parameters estimation based on
adaptive fitting modeling
GU Jia-hui,LU Feng, HUANG Jin-quan, QIANG Zi-jian
( Jiangsu Province Key Laboratory of Aerospace Power System, College of Energy &
Power Engineering,Nanjing University of Aeronautics and Astronautics,Nanjing 210016, China)
Abstract: Aiming at problem that components of aireraft engine will degrade slowly during its life cycle, but current linear model modeling methods of engine cannot meet requirement of precision for the whole life cycle, which leads to the fact that estimation errors of filtering algorithm for health parameters increases with flight cycles , an adaptive fitting method is proposed to establish state variable model( SVM ) and Kalman filter is designed for health parameters estimation. The method uses health parameter estimation value to realtime update related coefficient matrices in the last sampling period, so as to improve precision of the linear model. Linear variable parameter model is established for a commercial aircraft engine, at design cruise point on the basis of component level model. And by applying similarity transformation, at several flight state points, performance degradations process are digitally simulated. The simulation results reveal that KF based on the proposed modeling method greatly improves the estimation precision of health parameters and also has good real-time ability-
Keywords: commercial aircraft engine; gas path health parameters; state variable model ( SVM); Kalman filtering( KF) ; adaptive fitting modeling
0引言
航空发动机长期在高温、高压和高转速的恶劣环境中工作,会发生缓慢的性能退化现象,影响飞行安全[1.2]。通过收集商用航空发动机的机载传感器数据可以对发动机的状态进行监视与故障诊断[3-5],分析性能退化的程度帮助制定维修策略,从而提高飞行安全和降低航班延误率
航空发动机的气路健康状态常用各个部件的流量和效率的相对变化量(即健康参数)来表示,然而由于其无法直接测量,只能根据机载传感器的测量值,如两个转子转速和
收稿日期:2017-11-21
各个截面的压力和温度通过算法进行估计。常用的方法有各种滤波算法[5-8]。
在状态估计方面,卡尔曼滤波算法研究得较多[6-10],然而发动机部件级模型计算量较大,使得非线性滤波算法目前难以满足机载实时性要求,同时发动机线性状态变量模型(statevariablemodel,SVM)存在建模误差,使得线性卡尔受滤波算法对健康参数的估计精度较低。
常用的发动机状态变量模型建模方法有:扰动法")拟合法[12]、改进拟合法[13]等。但系数矩阵仅通过对发动
*基金项目:国家自然科学基金资助项目(51276087);国家自然科学基金青年科学基金资助项目(61304133)