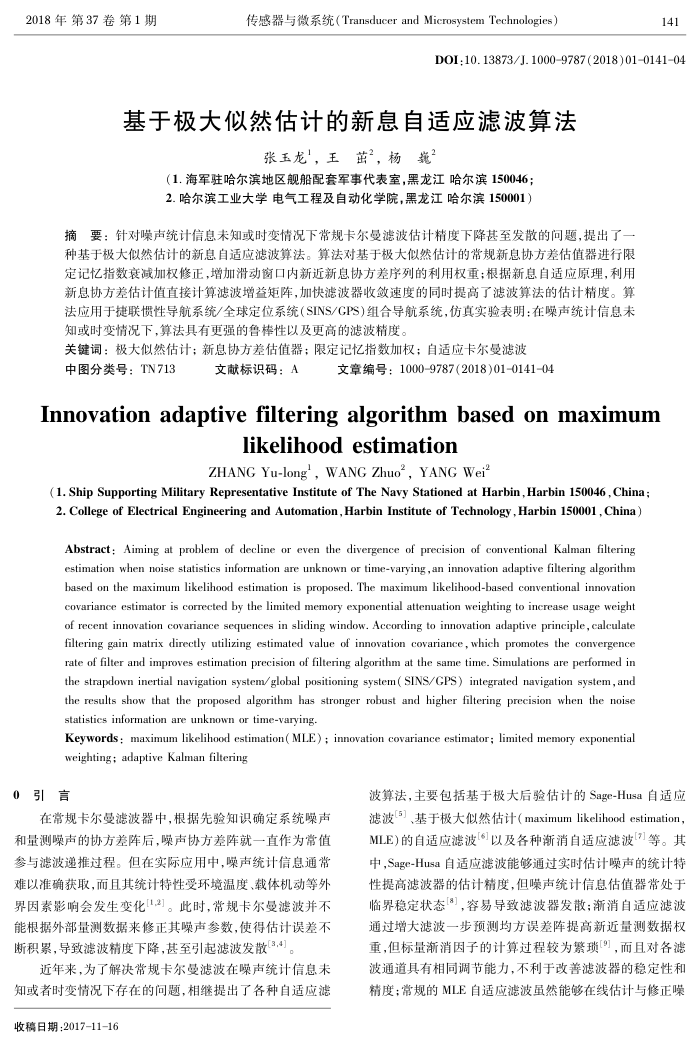
2018年第37卷第1期
传感器与微系统(Transducer andMicrosystemTechnologies)
141
DOI:10.13873/J.10009787(2018)01014104
基于极大似然估计的新息自适应滤波算法
张玉龙,王茁,杨巍
(1.海军驻哈尔滨地区舰船配套军事代表室,黑龙江哈尔滨150046: 2.哈尔滨工业大学电气工程及自动化学院,黑龙江哈尔滨150001)
摘要:针对噪声统计信息未知或时变情况下常规卡尔曼滤波估计精度下降甚至发散的问题,提出了一种基于极大似然估计的新息自适应滤波算法。算法对基于极大似然估计的常规新息协方差估值器进行限定记忆指数装减加权修正,增加滑动窗口内新近新息协方差序列的利用权重;根据新息自适应原理,利用新息协方差估计值直接计算滤波增益矩阵,加快滤波器收敛速度的同时提高了滤波算法的估计精度。算法应用于捷联惯性导航系统/全球定位系统(SINS/GPS)组合导航系统,仿真实验表明:在噪声统计信息未知或时变情况下,算法具有更强的鲁棒性以及更高的滤波精度,
关键词:极大似然估计;新息协方差估值器;限定记忆指数加权;自适应卡尔曼滤波
中图分类号:TN713
文献标识码:A
文章编号:1000-9787(2018)01-0141-04
Innovation adaptive filtering algorithm based on maximum
likelihoodestimation
ZHANG Yu-long',WANG Zhuo”,YANG Wei2
(1. Ship Supporting Military Representative Institute of The Navy Stationed at Harbin,Harbin 150046, China: 2. College of Electrical Engineering and Automation,Harbin Institute of Technology,Harbin 150001,China)
Abstract: Aiming at problem of decline or even the divergence of precision of conventional Kalman filtering estimation when noise statistics information are unknown or time-varying , an innovation adaptive filtering algorithm based on the maximum likelihood estimation is proposed. The maximum likelihood-based conventional innovation covariance estimator is corrected by the limited memory exponential attenuation weighting to increase usage weight of recent innovation covariance sequences in sliding window, According to innovation adaptive principle, calculate filtering gain matrix directly utilizing estimated value of innovation covariance, which promotes the convergence rate of filter and improves estimation precision of filtering algorithm at the same time. Simulations are performed in the strapdown inertial navigation system/global positioning system( SINS/GPS) integrated navigation system, and so a s a e eni aos eoe asor e s nsa statistics information are unknown or time-varying-
Keywords : maximum likelihood estimation( MLE) ; innovation covariance estimator; limited memory exponential weighting; adaptive Kalman filtering
0引言
在常规卡尔曼滤波器中,根据先验知识确定系统噪声和量测噪声的协方差阵后,噪声协方差阵就一直作为常值参与滤波递推过程。但在实际应用中,噪声统计信息通常难以准确获取,而且其统计特性受环境温度、载体机动等外界因素影响会发生变化[1,2]。此时,常规卡尔曼滤波并不能根据外部量测数据来修正其噪声参数,使得估计误差不断积累,导致滤波精度下降,甚至引起滤波发散(3.4]。
近年来,为了解决常规卡尔曼滤波在噪声统计信息未知或者时变情况下存在的问题,相继提出了各种自适应滤收稿日期:2017-11-16
波算法,主要包括基于极大后验估计的Sage-Husa自适应滤波5)基于极大似然估计(maximumlikelihoodestimation, MLE)的自适应滤波[]以及各种渐消自适应滤波汀等。其中,Sage-Husa自适应滤波能够通过实时估计噪声的统计特性提高滤波器的估计精度,但噪声统计信息估值器常处于临界稳定状态"],容易导致滤波器发散;渐消自适应滤波通过增大滤波一步预测均方误差阵提高新近量测数据权重,但标量渐消因子的计算过程较为繁琐9,而且对各滤波通道具有相同调节能力,不利于改善滤波器的稳定性和精度:常规的MLE自适应滤波虽然能够在线估计与修正噪