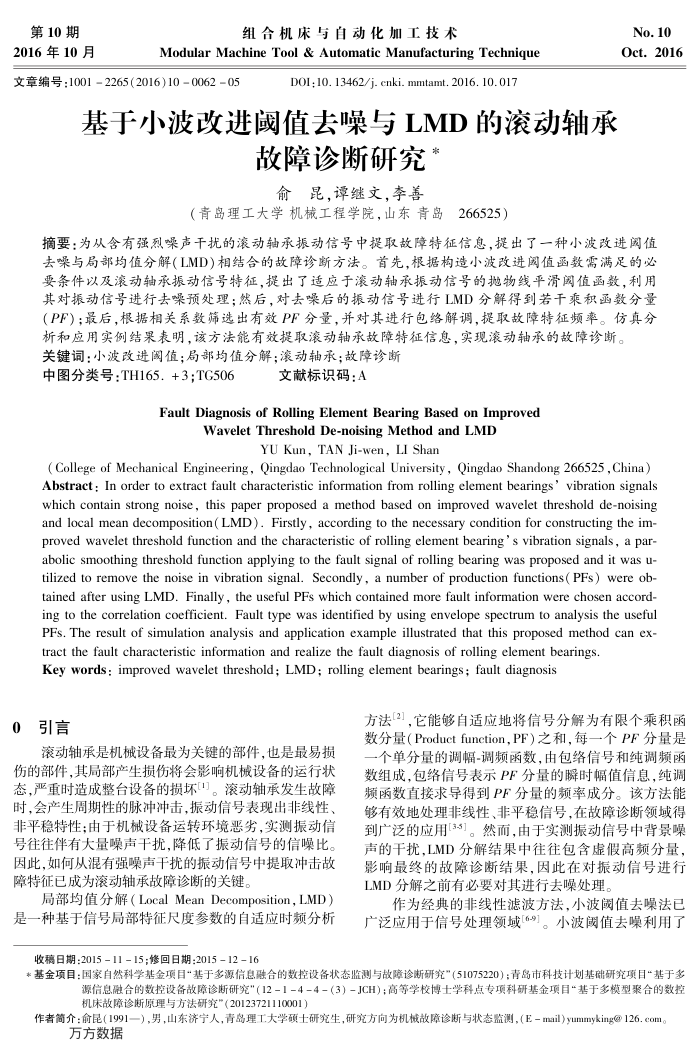
第10期 2016年10月
组合机床与自动化加工技术
ModularMachineTool&AutomaticManufacturingTechnique
文章编号:10012265(2016)10006205
D0I:10.13462/j.cnki.mmtamt.2016.10.017
基于小波改进阈值去噪与LMD的滚动轴承
故障诊断研究俞昆,谭继文,李善
(青岛理工大学机械工程学院,山东青岛266525)
No.10 Oct.2016
摘要:为从含有强烈噪声干扰的滚动轴承振动信号中提取故障特征信息,提出了一种小波改进阅值去噪与局部均值分解(LMD)相结合的故障诊断方法。首先,根据构造小波改进阅值函数需满足的必要条件以及滚动轴承振动信号特征,提出了适应于滚动轴承振动信号的抛物线平滑阅值函数,利用其对振动信号进行去噪预处理:然后,对去噪后的振动信号进行LMD分解得到若干乘积函数分量(PF);最后,根据相关系数筛选出有效PF分量,并对其进行包络解调,提取故障特征频率。仿真分
析和应用实例结果表明,该方法能有效提取滚动轴承故障特征信息,实现滚动轴承的故障诊断。关键词:小波改进阈值;局部均值分解:滚动轴承:故障诊断
中图分类号:TH165.+3;TG506
文献标识码:A
FaultDiagnosis ofRollingElementBearingBased onImproved
Wavelet Threshold De-noising Method and LMD
YU Kun, TAN Ji-wen, LI Shan
(College of Mechanical Engineering,Qingdao Technological University,Qingdao Shandong266525,China) Abstract: In order to extract fault characteristic information from rolling element bearings' vibration signals which contain strong noise, this paper proposed a method based on improved wavelet threshold de-noising and local mean decomposition(LMD). Firstly, according to the necessary condition for constructing the im-proved wavelet threshold function and the characteristic of rolling element bearing's vibration signals, a par-abolic smoothing threshold function applying to the fault signal of rolling bearing was proposed and it was u-tilized to remove the noise in vibration signal. Secondly, a number of production functions(PFs) were ob-tained after using LMD. Finally, the useful PFs which contained more fault information were chosen accord-ing to the correlation coefficient. Fault type was identified by using envelope spectrum to analysis the useful PFs. The result of simulation analysis and application example illustrated that this proposed method can ex-
tract the fault characteristic information and realize the fault diagnosis of rolling element bearings. Key words: improved wavelet threshold; LMD; rolling element bearings; fault diagnosis
0引言
滚动轴承是机械设备最为关键的部件,也是最易损伤的部件,其局部产生损伤将会影响机械设备的运行状态,严重时造成整台设备的损坏。滚动轴承发生故障时,会产生周期性的脉冲冲击,振动信号表现出非线性、非平稳特性:由于机械设备运转环境恶劣,实测振动信号往往伴有大量噪声干扰,降低了振动信号的信噪比。因此,如何从混有强噪声干扰的振动信号中提取冲击故障特征已成为滚动轴承故障诊断的关键。
局部均值分解(LocalMeanDecomposition,LMD)是一种基于信号局部特征尺度参数的自适应时频分析
收稿日期:2015-11-15;修回日期:2015-12-16
方法2],它能够自适应地将信号分解为有限个乘积函数分量(Productfunction,PF)之和,每一个PF分量是一个单分量的调幅-调频函数,由包络信号和纯调频函数组成,包络信号表示PF分量的瞬时幅值信息,纯调频函数直接求导得到PF分量的频率成分。该方法能够有效地处理非线性、非平稳信号,在故障诊断领域得到广泛的应用[3-5]。然而,由于实测振动信号中背景噪声的干扰,LMD分解结果中往往包含虚假高频分量,影响最终的故障诊断结果,因此在对振动信号进行 LMD分解之前有必要对其进行去噪处理。
作为经典的非线性滤波方法,小波阅值去噪法已广泛应用于信号处理领域[6-9]。小波阅值去噪利用了
*基金项目:国家自然科学基金项目“基于多源信息融合的数控设备状态监测与故障诊断研究"(51075220);青岛市科技计划基础研究项目“基于多
()1)机床故障诊断原理与方法研究"(20123721110001)
作者简介:俞昆(1991—),男,山东济宁人,青岛理工大学硕士研究生,研究方向为机械故障诊断与状态监测,(E-mail)yummyking@126.com。
方方数据