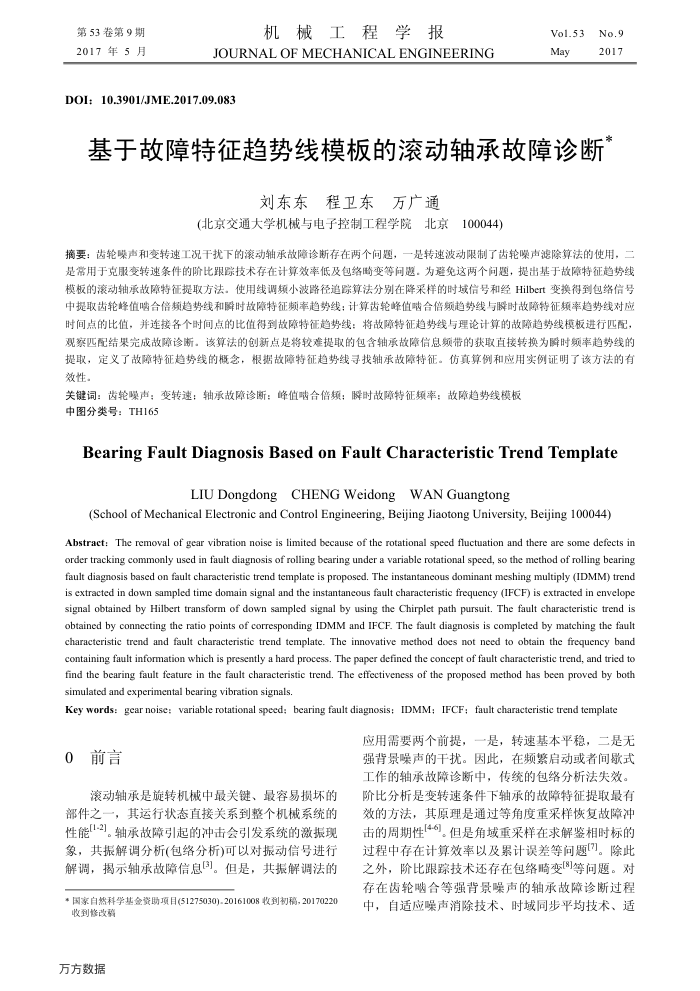
第53卷第9期 2017年5月
机械工程学报
JOURNALOFMECHANICALENGINEERING
DOI:10.3901/JME.2017.09.083
Vo1.53 May
No.9 2017
基于故障特征趋势线模板的滚动轴承故障诊断
刘东东程卫东万产通
(北京交通大学机械与电子控制工程学院北京100044)
模板的滚动轴承故障特征提取方法。使用线调频小波路径追踪算法分别在降采样的时域信号和经Hilbert变换得到包络信号中时间点的比值,并连接各个时间点的比值得到故障特征趋势线:将故障特征趋势线与理论计算的故障趋势线模板进行匹配,观察匹配结果完成故障诊断。该算法的创新点是将较难提取的包含轴承故障信息频带的获取直接转换为瞬时频率趋势线的提取,定义了故障特征趋势线的概念,根据故障特征趋势线寻找轴承故障特征。仿真算例和应用实例证明了该方法的有效性。
关键词:齿轮噪声:变转速:轴承故障诊断:峰值啮合倍频;瞬时故障特征频率:故障趋势线模板中图分类号:TH165
BearingFaultDiagnosisBasedonFaultCharacteristicTrendTemplate
LIUDongdongCHENG WeidongWAN Guangtong
(School of Mechanical Electronic and Control Engineering, Beijing Jiaotong University, Beijing 100044)
Abstract: The removal of gear vibration noise is limited because of the rotational speed fluctuation and there are some defects in order tracking commonly used in fault diagnosis of rolling bearing under a variable rotational speed, so the method of rolling bearing fault diagnosis based on fault characteristic trend template is proposed. The instantaneous dominant meshing multiply (IDMM) trend is extracted in down sampled time domain signal and the instantaneous fault characteristic frequency (IFCF) is extracted in envelope signal obtained by Hilbert transform of down sampled signal by using the Chirplet path pursuit. The fault characteristic trend is obtained by connecting the ratio points of corresponding IDMM and IFCF. The fault diagnosis is completed by matching the fault characteristic trend and fault characteristic trend template. The innovative method does not need to obtain the frequency band containing fault information which is presently a hard process. The paper defined the concept of fault characteristic trend, and tried t find the bearing fault feature in the fault characteristic trend. The effectiveness of the proposed method has been proved by both simulated and experimental bearing vibration signals.
Key words: gear noise: variable rotational speed: bearing fault diagnosis; IDMM; IFCF: fault characteristic trend templatc
0前言
滚动轴承是旋转机械中最关键、最容易损坏的部件之一,其运行状态直接关系到整个机械系统的性能[1-2]。轴承故障引起的冲击会引发系统的激振现象,共振解调分析(包络分析)可以对振动信号进行解调,揭示轴承故障信息[3]。但是,共振解调法的
*国家自然科学基金资助项目(51275030)-20161008收到初稿,20170220
收到修改整万方数据
应用需要两个前提,一是,转速基本平稳,二是无强背景噪声的干扰。因此,在频繁启动或者间歇式工作的轴承故障诊断中,传统的包络分析法失效。阶比分析是变转速条件下轴承的故障特征提取最有效的方法,其原理是通过等角度重采样恢复故障冲击的周期性[4-6]。但是角域重采样在求解鉴相时标的过程中存在计算效率以及累计误差等问题[}]。除此之外,阶比跟踪技术还存在包络畸变[8]等问题。对存在齿轮啮合等强背景噪声的轴承故障诊断过程中,自适应噪声消除技术、时域同步平均技术、适