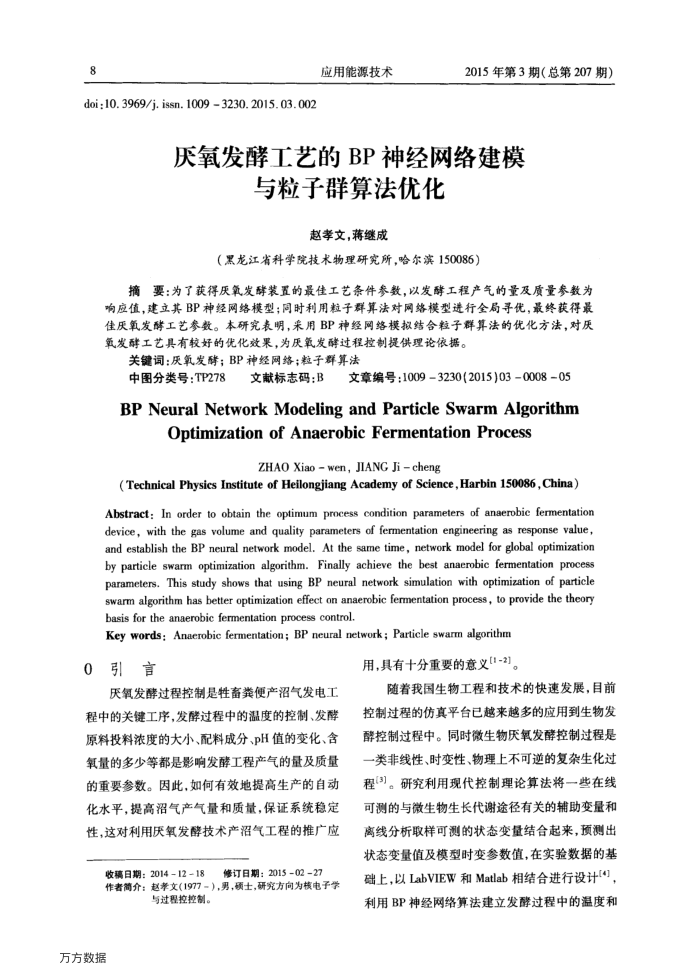
doi:10. 3969/j. issn. 1009 3230. 2015. 03.002
应用能源技术
2015年第3期(总第207期)
厌氧发酵工艺的BP神经网络建模
与粒子群算法优化
赵孝文,蒋继成
(黑龙江省科学院技术物理研究所,哈尔滨150086)
摘要:为了获得厌氧发酵装置的最佳工艺条件参数,以发酵工程产气的量及质量参数为响应值,建立其BP神经网络模型;同时利用粒子群算法对网络模型进行全局寻优,最终获得最佳厌氧发酵工艺参数。本研究表明,采用BP神经网络模拟结合粒子群算法的优化方法,对厌氧发酵工艺具有较好的优化效果,为厌氧发酵过程控制提供理论依据。
关键词:厌氧发酵;BP神经网络;粒子群算法
中图分类号:TP278
文章编号:10093230(2015)03000805
文献标志码:B
BP Neural Network Modeling and Particle Swarm Algorithm
OptimizationofAnaerobicFermentationProcess
ZHAO Xiao wen,JIANG Jicheng
(Technical Physics Institute of Heilongjiang Academy of Science, Harbin 150086, China) Abstract:In order to obtain the optimum process condition parameters of anaerobic fermentation device, with the gas volume and quality parameters of fermentation engineering as response value, and establish the BP neural network model, At the same time, network model for global optimization by particle swarm optimization algorithm.Finally achieve the best anaerobic fermentation process parameters. This study shows that using BP neural network simulation with optimization of particle swarm algorithm has better optimization effect on anaerobic fermentation process, to provide the theory basis for the anaerobic fermentation process control.
Key words: Anaerobic fermentation; BP neural network; Particle swarm algorithm
0引言
厌氧发酵过程控制是性畜粪便产沼气发电工程中的关键工序,发酵过程中的温度的控制、发酵原料投料浓度的大小、配料成分、pH值的变化、含氧量的多少等都是影响发酵工程产气的量及质量的重要参数。因此,如何有效地提高生产的自动化水平,提高沼气产气量和质量,保证系统稳定性,这对利用厌氧发酵技术产沼气工程的推广应
收稿日期:2014-12-18
修订日期:2015-0227
作者简介:赵举文(1977-),男,硕士,研究方向为核电子学
与过程控控制。
万方数据
用,具有十分重要的意义[1-2]。
随着我国生物工程和技术的快速发展,目前控制过程的仿真平台已越来越多的应用到生物发酵控制过程中。同时微生物厌氧发酵控制过程是一类非线性、时变性、物理上不可逆的复杂生化过程(3)。研究利用现代控制理论算法将一些在线可测的与微生物生长代谢途径有关的辅助变量和离线分析取样可测的状态变量结合起来,预测出状态变量值及模型时变参数值,在实验数据的基础上,以LabVIEW和Matlab相结合进行设计[4] 利用BP神经网络算法建立发酵过程中的温度和