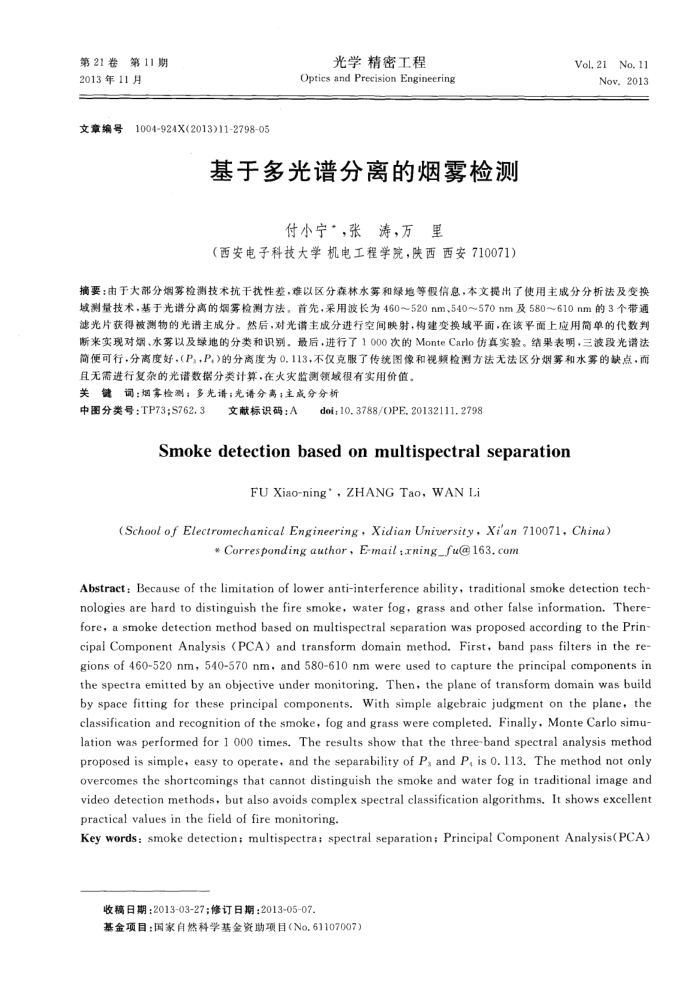
第11期
第21卷 2013年11月
文章编号
1004-924X(2013)11-2798-05
光学精密工程
Optics and Precision Engineering
基于多光谱分离的烟雾检测
付小宁,张涛,万里
(西安电子科技大学机电工程学院,陕西西安710071)
No.11
Vol.21
Nov.2013
摘要:由于大部分烟雾检测技术抗干扰性差,难以区分森林水雾和绿地等假信息,本文提出了使用主成分分析法及变换域测量技术,基于光谱分离的烟雾检测方法,首先,采用波长为460~520nm、540~570nm及580~610nm的3个带通滤光片获得被测物的光谱主成分。然后,对光谱主成分进行空间映射,构建变换域平面,在该平面上应用简单的代数判断来实现对烟、水雾以及绿地的分类和识别,最后,进行了1000次的MonteCarlo伤真实验。结果表明,三波段光谱法简便可行,分离度好,(Ps,P,>的分离度为0.113,不仅克服了传统图像和视频检测方法无法区分烟雾和水雾的缺点,而
且无需进行复杂的光谱数据分类计算,在火灾监测领域很有实用价值。关键词:烟雾检测;多光讲;光谱分离主成分分新
中图分类号:TP73;S762.3
文献标识码:A
doi;10,3788/OPE,20132111,2798
Smokedetectionbased onmultispectralseparation
FU Xiao-ning',ZHANG Tao,WANLi
(School of Electromechanical Engineering,XidianUniversity,Xi'an 710071,China)
Corresponding author,E-mail;ning_fu@163,com
Abstract: Because of the limitation of lower anti-interference ability, traditional smoke detection tech-nologies are hard to distinguish the fire smoke, water fog, grass and other false information. There-fore, a smoke detection method based on multispectral separation was proposed according to the Prin cipal Component Analysis (PCA) and transform domain method. First, band pass filters in the re-gions of 460-520 nm, 540-570 nm, and 580-610 nm were used to capture the principal components in the spectra emitted by an objective under monitoring. Then, the plane of transform domain was build by space fitting for these principal components. With simple algebraic judgment on the plane, the classification and recognition of the smoke, fog and grass were completed. Finally, Monte Carlo simu lation was performed for 1 ooo times. The results show that the three-band spectral analysis method proposed is simple, easy to operate, and the separability of Px and P, is o. 113. The method not only overcomes the shortcomings that cannot distinguish the smoke and water fog in traditional image and video detection methods, but also avoids complex spectral classification algorithms., It shows excellent practical values in the field of fire monitoring.
Key words: smoke detection; multispectra; spectral separation; Principal Component Analysis(PCA)
收稿日期:2013-03-27;修订日期:2013-05-07.
基金项目:国家自然科学基金资助项目(No.61107007)