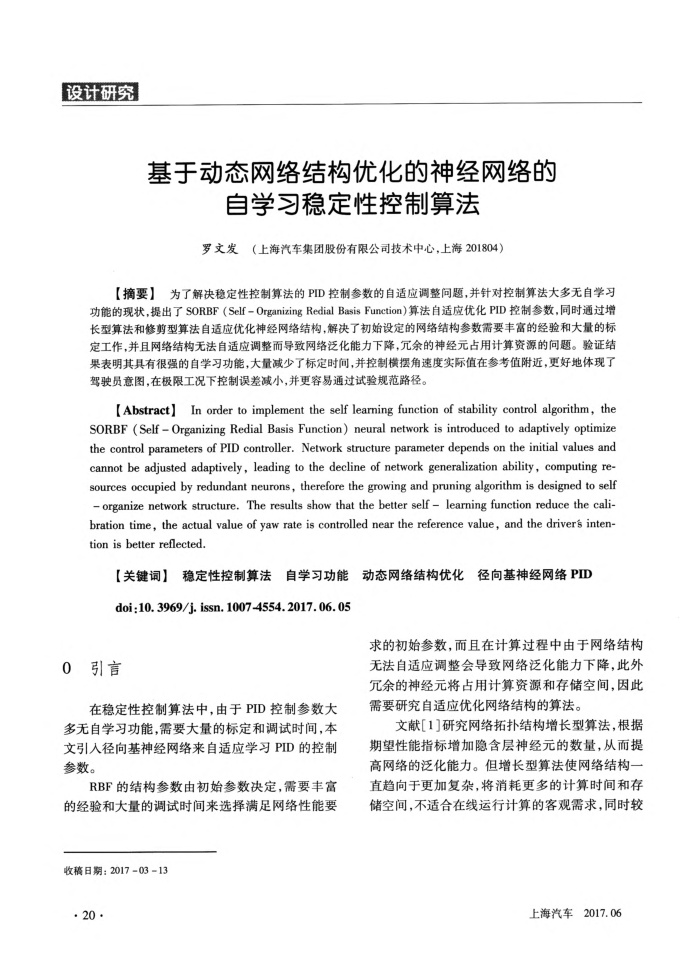
设计研究
基于动态网络结构优化的神经网络的
自学习稳定性控制算法
罗文发(上海汽车集团股份有限公司技术中心,上海201804)
【摘要】为了解决稳定性控制算法的PID控制参数的自适应调整间题,并针对控制算法大多无自学习功能的现状,提出了SORBF(Self-OrganizingRedialBasisFunction)算法自适应优化PID控制参数,同时通过增长型算法和修剪型算法自适应优化神经网络结构,解决了初始设定的网络结构参数需要丰富的经验和大量的标定工作,并且网络结构无法自适应调整而导致网络泛化能力下降,元余的神经元占用计算资源的问题。验证结果表明其具有很强的自学习功能,大量减少了标定时间,并控制横摆角速度实际值在参考值附近,更好地体现了驾驶员意图,在极限工况下控制误差减小,并更容易通过试验规范路径。
[Abstract]In order to implement the self learning function of stability control algorithm, the SORBF (Self Organizing Redial Basis Function) neural network is introduced to adaptively optimize the control parameters of PID controller. Network structure parameter depends on the initial values and cannot be adjusted adaptively, leading to the decline of network generalization ability, computing re-sources occupied by redundant neurons, therefore the growing and pruning algorithm is designed to self organize network structure. The results show that the better self learning function reduce the cali-bration time, the actual value of yaw rate is controlled near the reference value, and the drivers inten-tion is better reflected.
【关键词】稳定性控制算法自学习功能动态网络结构优化径向基神经网络PID doi:10.3969/j. issn.1007-4554.2017.06.05
求的初始参数,而且在计算过程中由于网络结构
0引言
在稳定性控制算法中,由于PID控制参数大多无自学习功能,需要大量的标定和调试时间,本文引入径向基神经网络来自适应学习PID的控制参数。
RBF的结构参数由初始参数决定,需要丰富的经验和大量的调试时间来选择满足网络性能要
收稿日期:2017-03-13· 20·
无法自适应调整会导致网络泛化能力下降,此外允余的神经元将占用计算资源和存储空间,因此需要研究自适应优化网络结构的算法。
文献[1]研究网络拓扑结构增长型算法,根据期望性能指标增加隐含层神经元的数量,从而提高网络的泛化能力。但增长型算法使网络结构直趋向于更加复杂,将消耗更多的计算时间和存储空间,不适合在线运行计算的客观需求,同时较
上海汽车2017.06