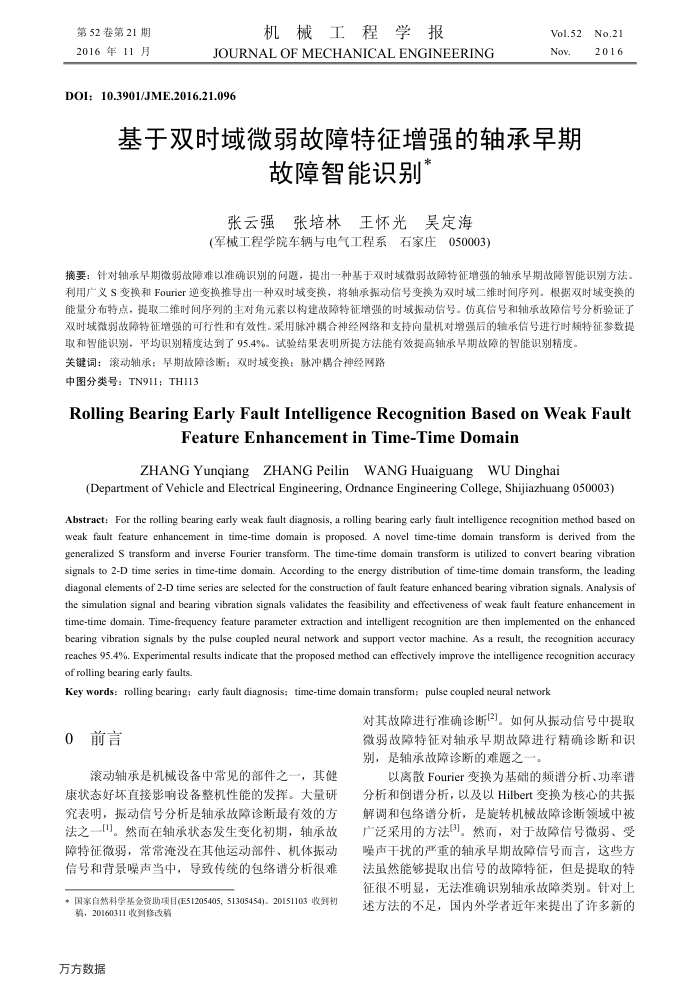
第52卷第21期 2016年11月
机械工程学报
JOURNALOFMECHANICALENGINEERING
DOI:10.3901/JME.2016.21.096
Vol.52 Nov.
基于双时域微弱故障特征增强的轴承早期
故障智能识别
张云强张培林王怀光吴定海
(军械工程学院车辆与电气工程系石家庄050003)
No.21 2016
摘要:针对轴承早期微弱故障难以准确识别的间题,提出一种基于双时域微弱故障待征增强的轴承早期故障智能识别方法。能量分布特点,提取二维时间序列的主对角元素以构建故障特征增强的时域振动信号。仿真信号和轴承故障信号分析验证了双时域微弱故障特征增强的可行性和有效性。采用脉冲耦合神经网络和支持向量机对增强后的轴承信号进行时频特征参数提取和智能识别,平均识别精度达到了95.4%。试验结果表明所提方法能有效提高轴承早期故障的智能识别精度。
关键词:滚动轴承:早期故障诊断:双时域变换:脉冲耦合神经网路中图分类号:TN911:TH113
RollingBearingEarlyFaultIntelligenceRecognitionBasedonWeakFault
FeatureEnhancementinTime-TimeDomain
ZHANGYunqiang
gZHANGPeilinWANGHuaiguang
gWUDinghai
(Department of Vehicle and Electrical Engineering, Ordnance Engineering College, Shijiazhuang 050003)
Abstraet: For the rolling bearing early weak fault diagnosis, a rolling bearing early fault intelligence recognition method based on weak fault feature enhancement in time-time domain is proposed. A novel time-time domain transform is derived from the generalized S transform and inverse Fourier transform. The time-time domain transform is utilized to convert bearing vibration signals to 2-D time series in time-time domain. According to the energy distribution of time-time domain transform, the leading diagonal elements of 2-D time series are selected for the construction of fault feature enhanced bearing vibration signals. Analysis of the simulation signal and bearing vibration signals validates the feasibility and effectiveness of weak fault feature enhancement in time-time domain. Time-frequency feature parameter extraction and intelligent recognition are then implemented on the enhanced bearing vibration signals by the pulse coupled neural network and support vector machine. As a result, the recognition accuracy ae of rolling bearing carly faults.
Key words: rolling bearing: carly fault diagnosis: time-time domain transform: pulse coupled neural network
对其故障进行准确诊断[]。如何从振动信号中提取
0
前言
滚动轴承是机械设备中常见的部件之一,其健
康状态好坏直接影响设备整机性能的发挥。大量研究表明,振动信号分析是轴承故障诊断最有效的方法之一[]。然而在轴承状态发生变化初期,轴承故障特征微弱,常常淹没在其他运动部件、机体振动信号和背景噪声当中,导致传统的包络谱分析很难
+国家自然科学基金资助项目(E51205405,51305454)。20151103收到初
稍,20160311收到修改障万方数据
微弱故障特征对轴承早期故障进行精确诊断和识别,是轴承故障诊断的难题之一。
以离散Fourier变换为基础的频谱分析、功率谱分析和倒谱分析,以及以Hilbert变换为核心的共振解调和包络谱分析,是旋转机械故障诊断领域中被广泛采用的方法③。然而,对于故障信号微弱、受噪声干扰的严重的轴承早期故障信号而言,这些方法虽然能够提取出信号的故障特征,但是提取的特征很不明显,无法准确识别轴承故障类别。针对上述方法的不足,国内外学者近年来提出了许多新的