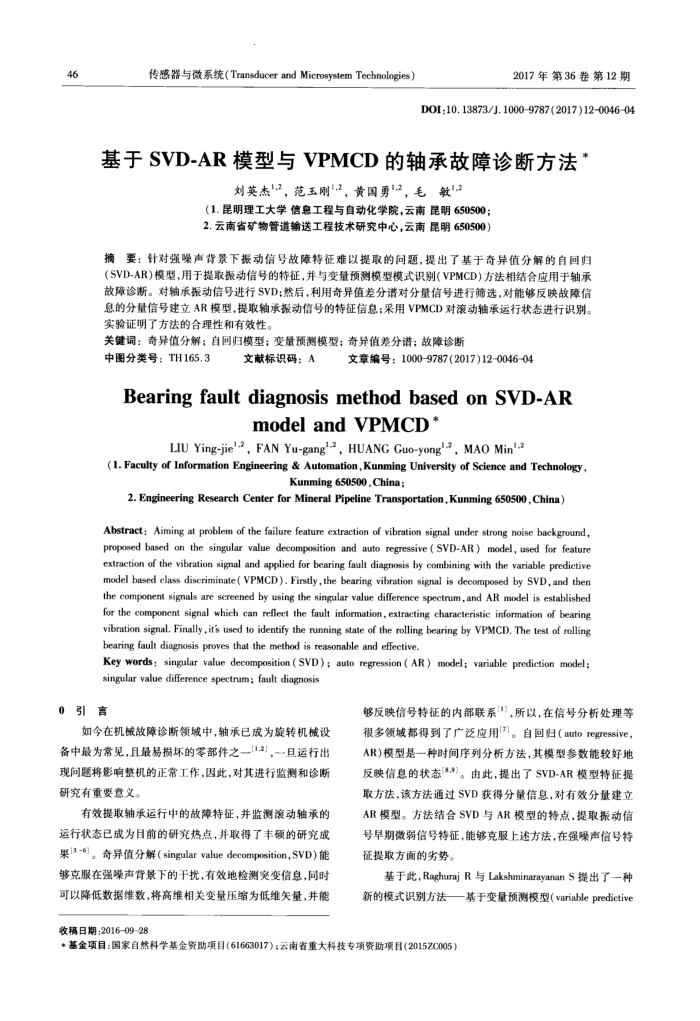
46
传感器与微系统(Transducerand MicrosystemTechnologies)
2017年第36卷第12期
DOI:10. 13873/J. 10009787(2017)12004604
基于SVD-AR模型与VPMCD的轴承故障诊断方法
刘英杰2,范玉刚"2,黄国勇12,毛敏"2
(1.昆明理工大学信息工程与自动化学院,云南昆明650500: 2.云南省矿物管道输送工程技术研究中心,云南昆明650500)
要:针对强噪声背景下振动信号故障特征难以提取的间题,提出了基于奇异值分解的自回归摄
(SVD-AR)模型,用于提取报动信号的特征,并与变量预测模型模式识别(VPMCD)方法相结合应用于轴承故障诊断。对轴承振动信号进行SVD;然后,利用奇异值差分谱对分量信号进行筛选,对能够反映故障信息的分量信号建立AR模型,提取轴承报动信号的特征信息;采用VPMCD对滚动轴承运行状态进行识别。实验证明了方法的合理性和有效性。
关键词:奇异值分解;自回归模型;变量预测模型;奇异值差分谱;故障诊断
中图分类号:TH165.3
文献标识码:A
文章编号:1000-9787(2017)12-0046-04
Bearing fault diagnosis method based
SVD-AR
on
modelandVPMCD
LIU Ying-je'-2, FAN Yu-gang"-2, HUANG Guo-yong"2, MAO Min'-2
(1. Faculty of Information Engineering & Automation,Kunming University of Science and Technology,
Kunming650500,China
2. Engineering Research Center for Mineral Pipeline Transportation,Kunming 650500, China)
Abstract: Aiming at problem of the failure feature extraction of vibration signal under strong noise background, aneay oy pesn po (VGAs ) aajssatia ot pue uogrsodtuooap aner sengus a uo peseq pasodoad extraction of the vibration signal and applied for bearing fault diagnosis by combining with the variable predictive model based elass diseriminate( VPMCD). Firstly,the bearing vibration signal is decomposed by SVD, and then the component signals are sereened by using the singular value difference spectrum, and AR model is established for the component signal which can reflect the fault information, extracting characteristic information of bearing vibration signal. Finally ,it's used to identify the running state of the rolling bearing by VPMCD. The test of rolling bearing fault diagnosis proves that the method is reasonable and effective.
Key words: singular value deeomposition (SVD); auto regression ( AR) model; variable prediection model; singular value difference spectrum; fault diagnosis
引言 0
如今在机械故障诊断领域中,轴承已成为旋转机械设备中最为常见,且最易损坏的零部件之一11.21,一旦运行出现问题将影响整机的正常工作,固此,对其进行监测和诊断研究有重要意义。
有效提取轴承运行中的故障特征,并监测滚动轴承的运行状态已成为目前的研究热点,并取得了丰硕的研究成果[3-6)。奇异值分解(singularvalue decomposition,SVD)能够克服在强噪声背景下的干扰,有效地检测突变信息,同时可以降低数据维数,将高维相关变量压缩为低维矢量,并能
收稿日期:2016-09-28
够反映信号特征的内部联系"),所以,在信号分析处理等很多领域都得到了广泛应用(")。自回归(autoregressive, AR)模型是一种时间序列分析方法,其模型参数能较好地反映信息的状态(*.")。由此,提出了SVD-AR模型特征提取方法,该方法通过SVD获得分量信息,对有效分量建立 AR模型。方法结合SVD与AR模型的特点,提取振动信号早期微弱信号特征,能够克服上述方法,在强噪声信号待征提取方面的劣势。
基于此,Raghuraj R与Lakshminarayanan S提出了种新的模式识别方法—基于变量预测模型(variablepredietive
*基金项目:国家自然科学基金资助项目(61663017):云南省重大科技专项资助项目(20157C005)