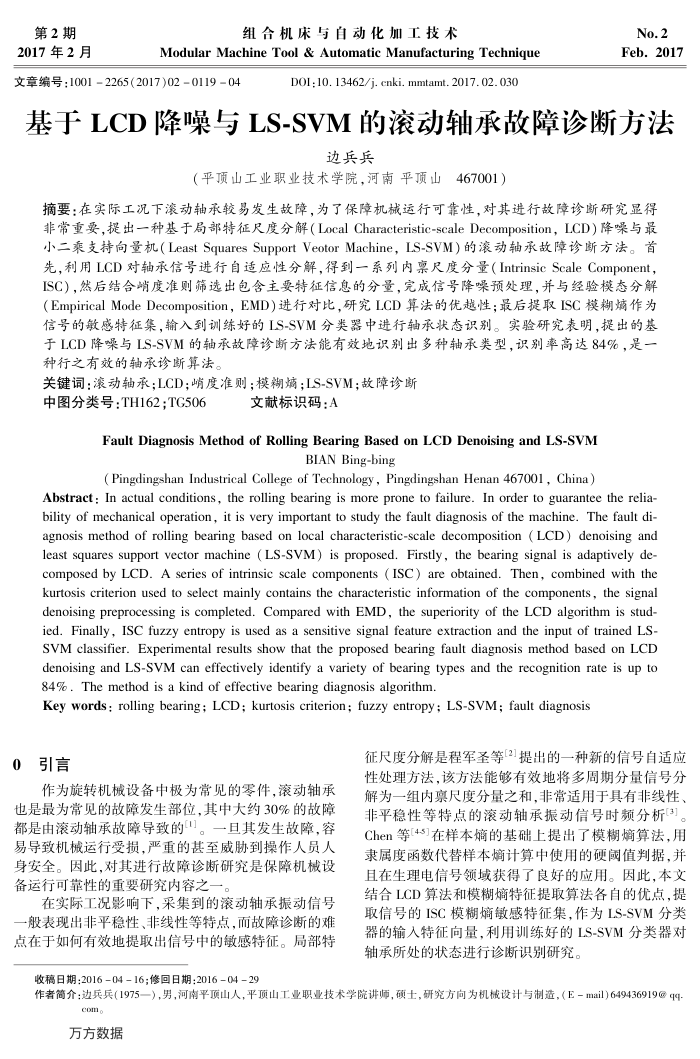
第2期 2017年2月
组合机床与自动化加工技术
ModularMachineTool&Automatic ManufacturingTechnique
文章编号:10012265(2017)02011904
D0I : 10. 13462/j. cnki. mmtamt. 2017. 02. 030
No.2 Feb.2017
基于LCD降噪与 LS-SVM的滚动轴承故障诊断方法
边兵兵
(平顶山工业职业技术学院,河南平顶山467001)
摘要:在实际工况下滚动轴承较易发生故障,为了保障机械运行可靠性,对其进行故障诊断研究显得非常重要,提出一种基于局部特征尺度分解(LocalCharacteristic-scaleDecomposition,LCD)降噪与最小二乘支持向量机(LeastSquaresSupportVeotorMachine,LS-SVM)的滚动轴承故障诊断方法。首先,利用LCD对轴承信号进行自适应性分解,得到一系列内案尺度分量(IntrinsicScaleComponent, ISC),然后结合峭度准则筛选出包含主要特征信息的分量,完成信号降噪预处理,并与经验模态分解(EmpiricalModeDecomposition,EMD)进行对比,研究LCD算法的优越性;最后提取ISC模糊作为信号的敏感特征集,输入到训练好的LS-SVM分类器中进行轴承状态识别。实验研究表明,提出的基于LCD降噪与LS-SVM的轴承故障诊断方法能有效地识别出多种轴承类型,识别率高达84%,是一种行之有效的轴承诊断算法。
关键词:滚动轴承;LCD;峭度准则;模糊;LS-SVM;故障诊断
中图分类号:TH162;TG506
文献标识码:A
Fault Diagnosis Method of Rolling Bearing Based on LCD Denoising and LS-SVM
BIANBing-bing
(Pingdingshan Industrical College of Technology, Pingdingshan Henan 467001, China)
Abstract: In actual conditions, the rolling bearing is more prone to failure. In order to guarantee the relia-bility of mechanical operation, it is very important to study the fault diagnosis of the machine. The fault di-agnosis method of rolling bearing based on local characteristic-scale decomposition (LCD) denoising and least squares support vector machine (LS-SVM) is proposed. Firstly, the bearing signal is adaptively de-composed by LCD. A series of intrinsic scale components (ISC) are obtained. Then, combined with the kurtosis criterion used to select mainly contains the characteristic information of the components, the signal denoising preprocessing is completed. Compared with EMD, the superiority of the LCD algorithm is stud-ied. Finally, ISC fuzzy entropy is used as a sensitive signal feature extraction and the input of trained LS-SVM classifier. Experimental results show that the proposed bearing fault diagnosis method based on LCD denoising and LS-SVM can effectively identify a variety of bearing types and the recognition rate is up to 84%. The method is a kind of effective bearing diagnosis algorithm.
Key words: rolling bearing; LCD; kurtosis criterion; fuzzy entropy; LS-SVM; fault diagnosis
0引言
作为旋转机械设备中极为常见的零件,滚动轴承也是最为常见的故障发生部位,其中大约30%的故障都是由滚动轴承故障导致的"。一旦其发生故障,容易导致机械运行受损,严重的甚至威胁到操作人员人身安全。因此,对其进行故障诊断研究是保障机械设备运行可靠性的重要研究内容之一。
在实际工况影响下,采集到的滚动轴承振动信号一般表现出非平稳性、非线性等特点,而故障诊断的难点在于如何有效地提取出信号中的敏感特征。局部特
征尺度分解是程军圣等[2提出的一种新的信号自适应性处理方法,该方法能够有效地将多周期分量信号分解为一组内烹尺度分量之和,非常适用于具有非线性、非平稳性等特点的滚动轴承振动信号时频分析[3]。 Chen等[45]在样本嫡的基础上提出了模糊炳算法,用隶属度函数代替样本熵计算中使用的硬阈值判据,并且在生理电信号领域获得了良好的应用。因此,本文结合LCD算法和模糊嫡特征提取算法各自的优点,提取信号的ISC模糊嫡敏感特征集,作为LS-SVM分类器的输入特征向量,利用训练好的LS-SVM分类器对轴承所处的状态进行诊断识别研究。
收稿日期:2016-04-16修回日期:2016-04-29
作者简介:边兵兵(1975—),男,河南平项山人,平项山工业职业技术学院讲师,硕士,研究方向为机械设计与制造,(E-mail)649436919@qq
com。
万方数据