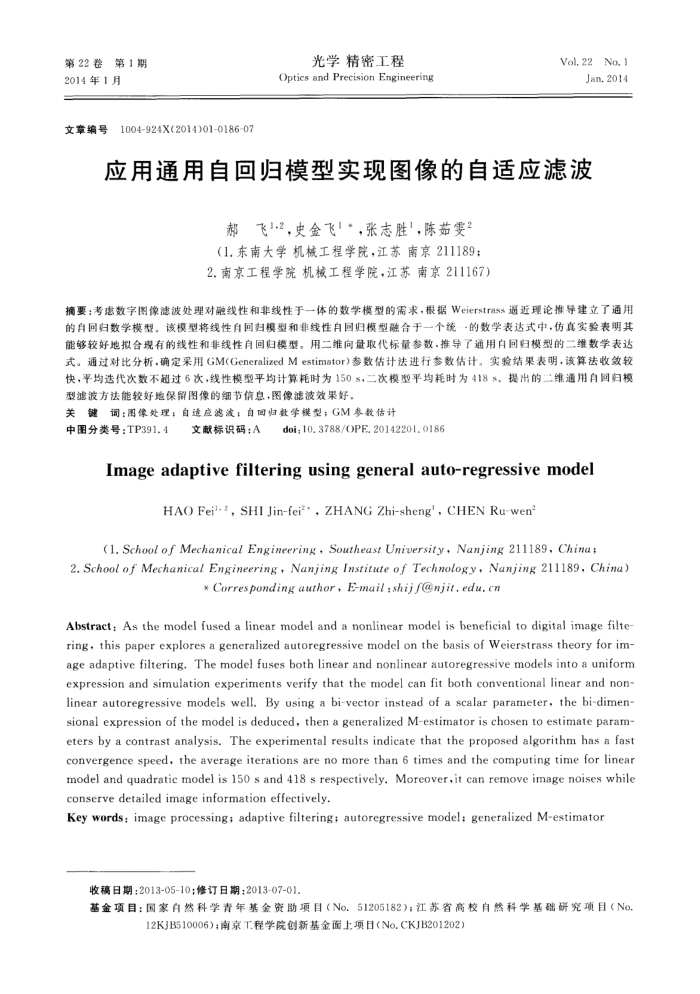
第22卷第1期 2014年1月
文章编号
1004-924X(2014)01018607
光学精密工程
Optics and Precision Engineering
Vol. 22No. 1
Jan.2014
应用通用自回归模型实现图像的自适应滤波
郝飞1.2,史金飞*,张志胜",陈茹雯2(1.东南大学机械工程学院,江苏南京211189: 2.南京工程学院机械工程学院,江苏南京211167)
摘要:考虑数字图像滤波处理对融线性和非线性于一体的数学模型的需求,根据Weierstrass逼近理论推导建立了通用的自回归数学模型。该模型将线性自回归模型和非线性自回归模型融合于一个统-的数学表达式中,仿真实验表明其能够较好地拟合现有的线性和非线性自回归模型。用二维向量取代标量参数,推导了通用白回归模型的二维数学表达式。通过对比分析,确定采用GM(Generalized Mestimator)参数估计法进行参数估计。实验结果表明,该算法收敛较快,平均选代次数不超过6次,线性模型平均计算耗时为150s,二次模型平均耗时为4183。提出的二维通用自回归模型滤波方法能较好地保留图像的细节信息,图像滤波效果好。
关键调:图像处理,自适应滤波;自回归数学模型;GM参数估计
中图分类号:TP391.4
文献标识码:A
doi;10, 3788/(PE, 20142201. 0186
Imageadaptivefilteringusinggeneral auto-regressivemodel
HAO Feil., SHI Jin-fei?, ZHANG Zhi-sheng', CHEN Ru-wen
(1.School of Mechanical Engineering,Southeast University,Nanjing 211189,Chind
2.School of Mechanical Engineering,Nanjing Institute of Technology,Nanjing 211189, China)
Corresponding author,E-mail :shijf@njit.edu.cn
Abstract: As the model fused a linear model and a nonlinear model is beneficial to digital image filte ring, this paper explores a generalized autoregressive model on the basis of Weierstrass theory for im age adaptive filtering. The model fuses both linear and nonlinear autoregressive models into a uniform expression and simulation experiments verify that the model can fit both conventional linear and non-linear autoregressive models well. By using a bi-vector instead of a scalar parameter, the bi-dimen sional expression of the model is deduced, then a generalized M-estimator is chosen to estimate param-eters by a contrast analysis. The experimental results indicate that the proposed algorithm has a fast convergence speed, the average iterations are no more than 6 times and the computing time for linear model and quadratic model is 150 s and 418 s respectively. Moreover,it can remove image noises while conserve detailed image information effectively.
Key words: image processing; adaptive filtering; autoregressive model; generalized M-estimator
收稿日期:2013-05-10:修订日期:2013-07-01.
基金项目:国家自然科学青年基金资助项目(No.51205182);江苏省高校自然科学基础研究项目(No.
12KJB510006);南京.T.程学院创新基金面上项日(No.CKJB201202)