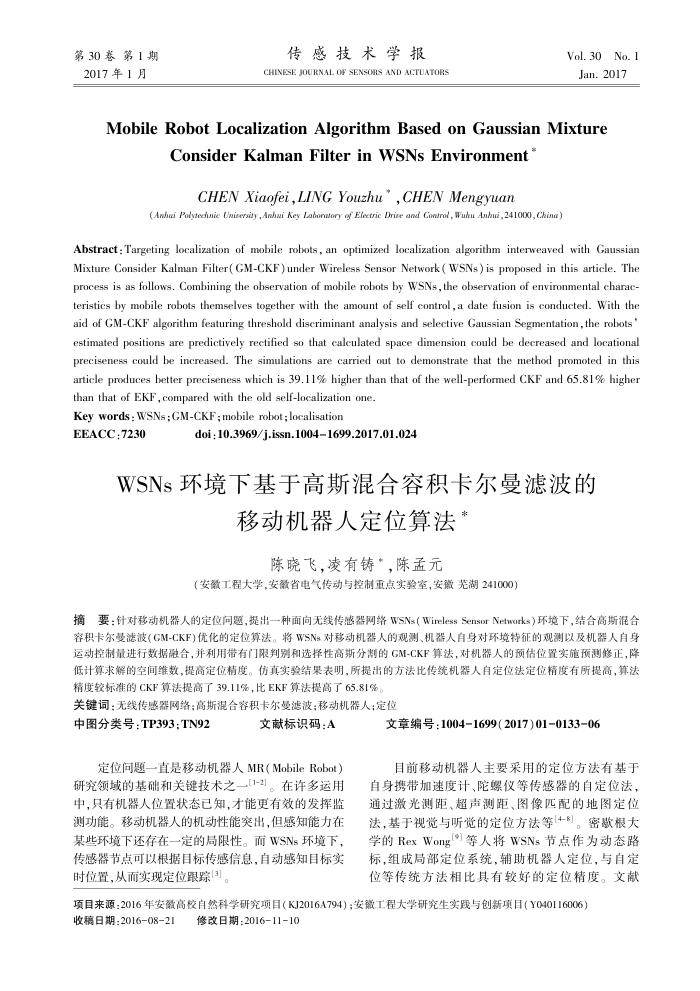
第30第1期 2017年1月
传感技术学报
CHINESE JOURNAL OF SENSORS AND ACTUATORS
Vol. 30No. 1 Jan.2017
MobileRobotLocalizationAlgorithmBasedonGaussianMixture
ConsiderKalmanFilterinWSNsEnvironment
CHEN Xiaofei,LING Youzhu,CHEN Mengyuan
(Anhui Polytechnic /nizersily,Anhai Key Labonatlory of Electric Drnize and Gontrol, Wuhu Ankui,241000, China)
Abstract: Targeting localization of mobile robots, an optimized localization algorithm interweaved with Gaussian Mixture Consider Kalman Filter( GM-CKF) under Wireless Sensor Network ( WSNs ) is proposed in this article. The process is as follows. Combining the observation of mobile robots by WSNs , the observation of environmental charac-teristics by mobile robots themselves together with the amount of self control, a date fusion is conducted. With the aid of GM-CKF algorithm featuring threshold discriminant analysis and selective Gaussian Segmentation,the robots estimated positions are predictively rectified so that caleulated space dimension could be decreased and locational preciseness could be increased. The simulations are carried out to demonstrate that the method promoted in this article produces better preciseness which is 39.11% higher than that of the well-performed CKF and 65.81% higher
than that of EKF, compared with the old self-localization one. Key words : WSNs ; GM-CKF ; mobile robot ; localisation
EEACC:7230
doi:10.3969/j.issn.10041699.2017.01.024
WSNs环境下基于高斯混合容积卡尔曼滤波的
移动机器人定位算法*
陈晓飞,凌有铸*,陈孟元
(安徽工程大学,安徽省电气传动与控制重点实验室,安徽芜湖241000)
摘要:针对移动机器人的定位间题,提出一种面向无线传感器网络WSNs(WirelessSensorNetworks)环境下,结合高斯混合容积卡尔曼滤波(GM-CKF)优化的定位算法。将WSNs对移动机器人的观测、机器人自身对环境特征的观测以及机器人自身运动控制量进行数据融合,并利用带有门限判别和选择性高斯分制的GM-CKF算法,对机器人的预估位置实施预测修正,降低计算求解的空间维数,提高定位精度。伤真实验结果表明,所提出的方法比传统机器人自定位法定位精度有所提高,算法
精度较标准的CKF算法提高了39.11%,比EKF算法提高了65.81%。关键词:无线传感器网络;高斯混合容积卡尔曼滤波;移动机器人;定位
中图分类号:TP393:TN92
文献标识码:A
定位间题一直是移动机器人MR(MobileRobot)研究领域的基础和关键技术之一[1-2]。在许多运用中,只有机器人位置状态已知,才能更有效的发挥监测功能。移动机器人的机动性能突出,但感知能力在某些环境下还存在一定的局限性。而WSNs环境下,传感器节点可以根据目标传感信息,自动感知目标实时位置,从而实现定位跟踪3」。
文章编号:1004-1699(2017)01-0133-06
目前移动机器人主要采用的定位方法有基于自身携带加速度计、陀螺仪等传感器的自定位法,通过激光测距、超声测距、图像匹配的地图定位法,基于视觉与听觉的定位方法等[4-8]。密歇根大学的RexWong]等人将WSNs节点作为动态路标,组成局部定位系统,辅助机器人定位,与自定位等传统方法相比具有较好的定位精度。文献
项目来源:2016年安徽高校自然科学研究项目(KJ2016A794);安徽工程大学研究生实践与创新项目(Y040116006)
收稿日期:2016-08-21
修改日期:2016-11-10