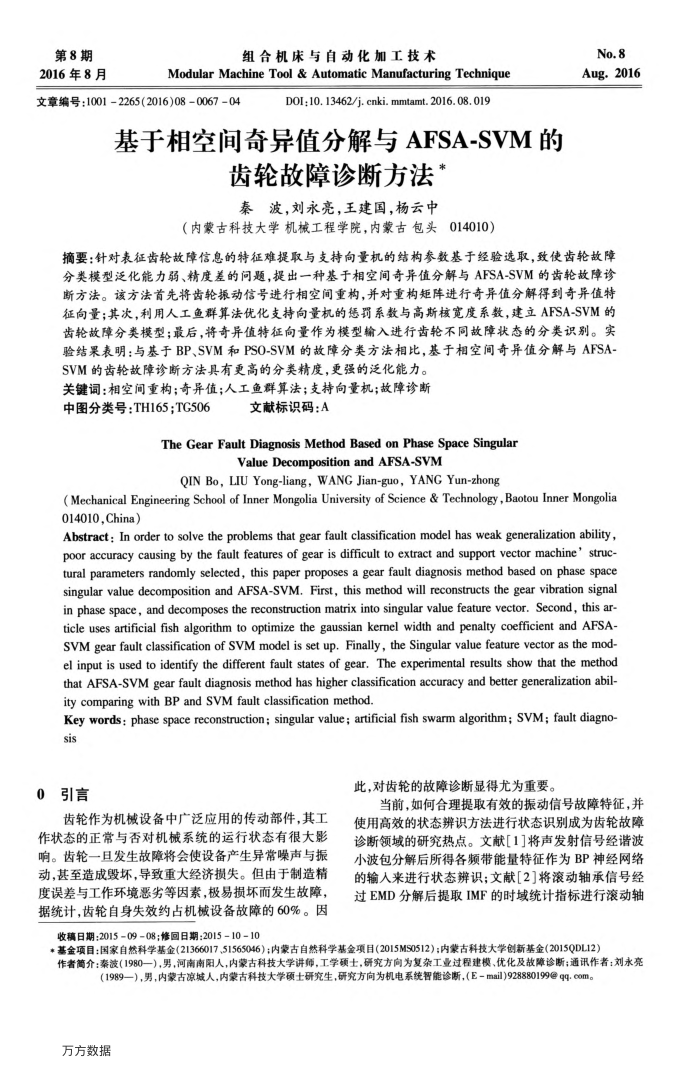
第8期 2016年8月
组合机床与自动化加工技术
ModularMachineTool&AutomaticManufacturingTechnique
文章编号:10012265(2016)08006704
DOI:10.13462/j. cnki. mmtamt.2016.08.019
基于相空间奇异值分解与AFSA-SVM的
齿轮故障诊断方法秦波,刘永亮,王建国,杨云中
(内蒙古科技大学机械工程学院,内蒙古包头014010)
No.8 Aug.2016
摘要:针对表征齿轮故障信息的特征难提取与支持向量机的结构参数基于经验选取,致使齿轮故障分类模型泛化能力弱、精度差的问题,提出一种基于相空间奇异值分解与AFSA-SVM的齿轮故障诊断方法。该方法首先将齿轮振动信号进行相空间重构,并对重构矩阵进行奇异值分解得到奇异值特征向量;其次,利用人工鱼群算法优化支持向量机的惩罚系数与高斯核宽度系数,建立AFSA-SVM的齿轮故障分类模型;最后,将奇异值特征向量作为模型输入进行齿轮不同故障状态的分类识别。实验结果表明:与基于BP、SVM和PSO-SVM的故障分类方法相比,基于相空间奇异值分解与AFSA
SVM的齿轮故障诊断方法具有更高的分类精度,更强的泛化能力。关键词:相空间重构;奇异值;人工鱼群算法;支持向量机;故障诊断
中图分类号:TH165;TG506
文献标识码:A
The Gear Fault Diagnosis Method Based on Phase Space Singular
Value Decomposition and AFSA-SVM
QIN Bo, LIU Yong-liang, WANG Jian-guo, YANG Yun-zhong
( Mechanical Engineering School of Inner Mongolia University of Science & Technology , Baotou Inner Mongolia 014010,China)
Abstract: In order to solve the problems that gear fault classification model has weak generalization ability, poor accuracy causing by the fault features of gear is difficult to extract and support vector machine' struc-tural parameters randomly selected, this paper proposes a gear fault diagnosis method based on phase space singular value decomposition and AFSA-SVM. First, this method will reconstructs the gear vibration signal in phase space, and decomposes the reconstruction matrix into singular value feature vector. Second, this ar-ticle uses artificial fish algorithm to optimize the gaussian kernel width and penalty coefficient and AFSA SVM gear fault classification of SVM model is set up. Finally, the Singular value feature vector as the mod-el input is used to identify the different fault states of gear. The experimental results show that the method that AFSA-SVM gear fault diagnosis method has higher classification accuracy and better generalization abil ity comparing with BP and SVM fault classification method.
Key words: phase space reconstruction; singular value; artificial fish swarm algorithm; SVM; fault diagno sis
0引言
齿轮作为机械设备中广泛应用的传动部件,其工作状态的正常与否对机械系统的运行状态有很大影响。齿轮一且发生故障将会使设备产生异常噪声与振动,基至造成毁坏,导致重大经济损失。但由于制造精度误差与工作环境恶劣等因素,极易损坏而发生故障,据统计,齿轮自身失效约占机械设备故障的60%。因
此,对齿轮的故障诊断显得尤为重要。
当前,如何合理提取有效的振动信号故障特征,并使用高效的状态辨识方法进行状态识别成为齿轮故障诊断领域的研究热点。文献[1]将声发射信号经谐波小波包分解后所得各频带能量特征作为BP神经网络的输人来进行状态辨识;文献【2]将滚动轴承信号经过EMD分解后提取IMF的时域统计指标进行滚动轴
收稿日期:201509-08;修回日期:2015-10-10
*基全项目:国家自然科学基金(21366017、51565046);内蒙古自然科学基金项目(2015MS0512);内蒙古科技大学创新基金(2015QDL12)
作者简介:秦波(1980一),男,河南南阳人,内蒙古科技大学讲师,工学硕士,研究方向为复杂工业过程建模、优化及故障诊断;通讯作者;刘永亮
(1989—),男,内蒙古凉城人,内蒙古科技大学硕士研究生,研究方向为机电系统智能诊断,(E-mail)928880199@qq.com。
万方数据