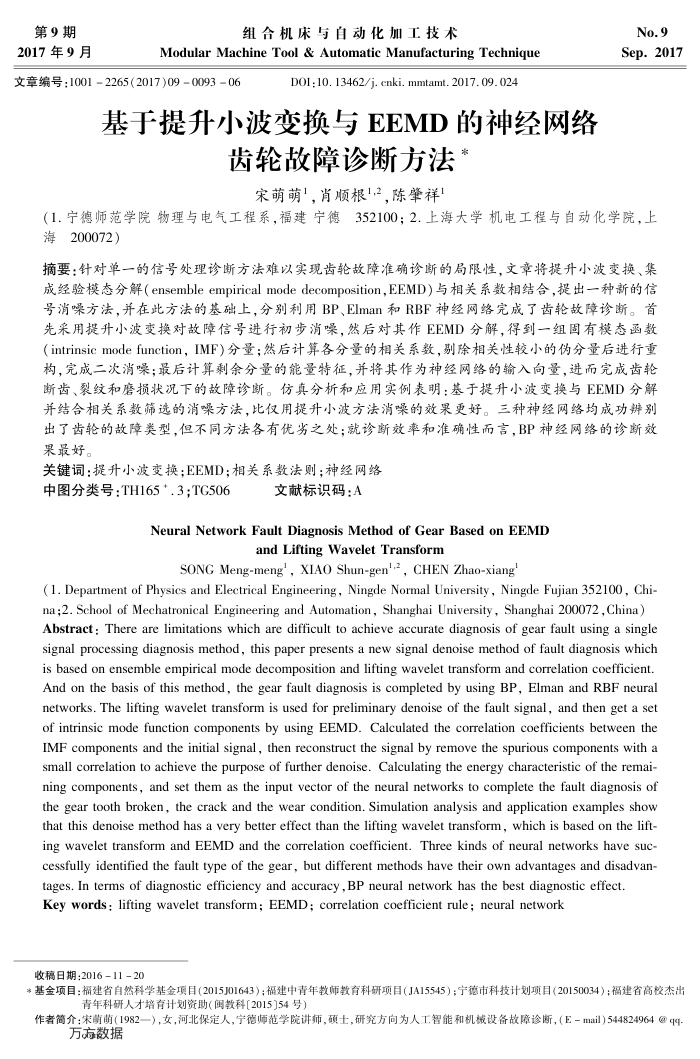
第9期 2017年9月
组合机床与自动化加工技术
ModularMachineTool&AutomaticManufacturingTechnique
文章编号:10012265(2017)09009306
D0I:10.13462/j.cnki.mmtamt.2017.09.024
基于提升小波变换与EEMID的神经网络
齿轮故障诊断方法宋萌萌1,肖顺根1,2,陈肇祥
No.9 Sep.2017
(1.宁德师范学院物理与电气工程系,福建宁德352100;2.上海大学机电工程与自动化学院,上海200072)
摘要:针对单一的信号处理诊断方法难以实现齿轮故障准确诊断的局限性,文章将提升小波变换、集成经验模态分解(ensembleempiricalmodedecomposition,EEMD)与相关系数相结合,提出一种新的信号消噪方法,并在此方法的基础上,分别利用BP、Elman和RBF神经网络完成了齿轮故障诊断。首先采用提升小波变换对故障信号进行初步消噪,然后对其作EEMD分解,得到一组固有模态函数(intrinsicmodefunction,IMF)分量;然后计算各分量的相关系数,剔除相关性较小的伪分量后进行重构,完成二次消噪;最后计算剩余分量的能量特征,并将其作为神经网络的输入向量,进而完成齿轮断齿、裂纹和磨损状况下的故障诊断。仿真分析和应用实例表明:基于提升小波变换与EEMD分解并结合相关系数筛选的消噪方法,比仅用提升小波方法消噪的效果更好。三种神经网络均成功辨别出了齿轮的故障类型,但不同方法各有优劣之处;就诊断效率和准确性而言,BP神经网络的诊断效果最好。
关键词:提升小波变换:EEMD:相关系数法则;神经网络
中图分类号:TH165+.3;TG506
文献标识码:A
Neural NetworkFault Diagnosis Method of GearBased onEEMD
and Lifting Wavelet Transform
SONG Meng-meng', XIAO Shun-gen'2, CHEN Zhao-xiang
(1. Department of Physics and Electrical Engineering, Ningde Normal University, Ningde Fujian 352100, Chi-na ;2. School of Mechatronical Engineering and Automation, Shanghai University, Shanghai 200072,China) Abstract: There are limitations which are difficult to achieve accurate diagnosis of gear fault using a single signal processing diagnosis method, this paper presents a new signal denoise method of fault diagnosis which is based on ensemble empirical mode decomposition and lifting wavelet transform and correlation coefficient And on the basis of this method, the gear fault diagnosis is completed by using BP, Elman and RBF neural networks. The lifting wavelet transform is used for preliminary denoise of the fault signal, and then get a set of intrinsic mode function components by using EEMD. Calculated the correlation coefficients between the IMF components and the initial signal,then reconstruct the signal by remove the spurious components with a small correlation to achieve the purpose of further denoise. Calculating the energy characteristic of the remai-ning components, and set them as the input vector of the neural networks to complete the fault diagnosis of the gear tooth broken, the crack and the wear condition. Simulation analysis and application examples show that this denoise method has a very better effect than the lifting wavelet transform, which is based on the lift-ing wavelet transform and EEMD and the correlation coefficient. Three kinds of neural networks have suc-cessfully identified the fault type of the gear, but different methods have their own advantages and disadvan tages. In terms of diagnostic efficiency and accuracy,BP neural network has the best diagnostic effect.
Key words: lifting wavelet transform; EEMD; correlation coefficient rule; neural network 收稿日期:2016-11-20
*基金项目:福建省自然科学基金项目(2015J01643);福建中青年教师教育科研项目(JA15545);宁德市科技计划项目(20150034);福建省高校杰出
青年科研人才培育计划资助(闽教科【2015]54号)
作者简介:宋菌萌(1982—),女,河北保定人,宁德师范学院讲师,硕士,研究方向为人工智能和机械设备故障诊断,(E-mail)544824964③q
万方数据