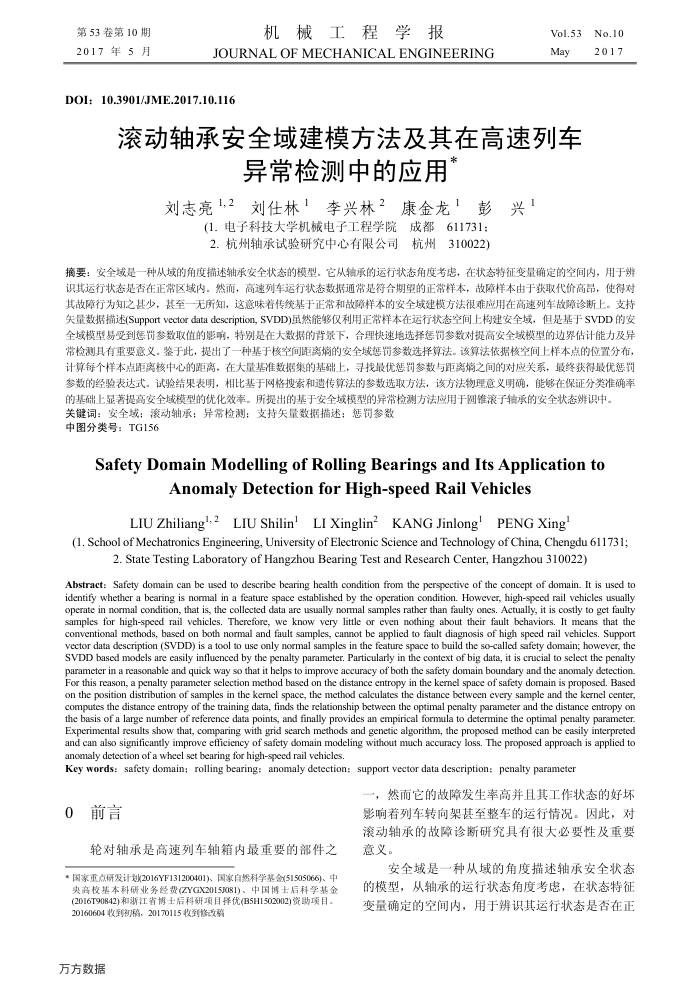
第53卷第10期 2017年5月
机械工程学报
JOURNALOF MECHANICALENGINEERING
DOI:10.3901/JME.2017.10.116
No.10
Vol.53
May
滚动轴承安全域建模方法及其在高速列车
异常检测中的应用
刘志亮1,2刘仕林1李兴林2康金龙1彭兴!
(1.电子科技大学机械电子工程学院成都611731: 2.杭州轴承试验研究中心有限公司杭州310022)
2017
摘要:安全域是一种从域的角度描述轴承安全状态的模型。它从轴承的运行状态角度考患,在状态特征变量确定的空间内,用于辨识其运行状态是否在正常区域内。然而,高速列车运行状态数据通常是符合期望的正常样本,故障样本由于获取代价高昂,使得对其故障行为知之甚少,甚至一无所知,这意味着传统基于正常和故障样本的安全域建模方法很难应用在高速列车故障诊断上。支持矢量数据描述(Supportvectordata description,SVDD)虽然能够仅利用正常样本在运行状态空间上构建安全域,但是基于SVDD的安全域模型易受到惩罚参数取值的影响,特别是在大数据的背景下,合理快速地选择惩罚参数对提高安全域模型的边界估计能力及异
计算每个样本点距离核中心的距离,在大量基准数据集的基础上,寻找最优惩罚参数与距离摘之间的对应关系,最终获得最优惩罚参数的经验表达式。试验结果表明,相比基于网格搜案和遗传算法的参数选取方法,该方法物理意义明确,能够在保证分类准确率的基础上显著提高安全域模型的优化效率。所提出的基于安全域模型的异常检测方法应用于圆维滚子轴承的安全状态辨识中,
关键词:安全域:滚动轴承;异常检测:支持矢量数据描述;惩罚参数中图分类号:TG156
SafetyDomain Modelling of Rolling Bearings and Its Application to
AnomalyDetectionforHigh-speedRailVehicles
LIU Zhiliang',2 LIU Shilin' LI Xinglin? KANG Jinlong' PENG Xing"
(1. School of Mechatronics Engineering, University of Electronic Science and Technology of China, Chengdu 611731;
2. State Testing Laboratory of Hangzhou Bearing Test and Research Center, Hangzhou 310022)
Abstract: Safety domain can be used to describe bearing health condition from the perspective of the concept of domain. It is used to identify whether a bearing is normal in a feature space established by the operation condition. However, high-speed rail vehicles usually operate in normal condition, that is, the collected data are usually normal samples rather than faulty ones. Actually, it is costly to get faulty samples for high-speed rail vehicles. Therefore, we know very little or even nothing about their fault behaviors. It means that the odnssaara ei paads o sisop e o pode aq oeasaes e pe eu oq o ae spoa eoaa vector data description (SVDD) is a tool to use only normal samples in the feature space to build the so-called safety domain; however, the SVDD based models are easily influenced by the penalty parameter. Particularly in the context of big data, it is crucial to select the penalty parameter in a reasonable and quick way so that it helps to improve accuracy of both the safety domain boundary and the anomaly detection For this reason, a penalty parameter selection method based on the distance entropy in the kermel space of safety domain is proposed. Based on the position distribution of samples in the kemel space, the method calculates the distance between every sample and the kernel center, computes the distance entropy of the training data, finds the relationship between the optimal penalty parameter and the distance entropy on the basis of a large number of reference data points, and finaly provides an empirical formula to determine the optimal penalty parameter. Experimental results show that, comparing with grid search methods and genetic algorithm, the proposed method can be easily interpreted and can also significantly improve efficiency of safety domain modeling without much accuracy loss. The proposed approach is applied to anomaly detection of a wheel set bearing for high-speed rail vehicles.
Key words: safety domain; rolling bearing: anomaly detection: support vector data description: penalty parameter
一,然而它的故障发生率高并且其工作状态的好坏
0
前言
轮对轴承是高速列车轴箱内最重要的部件之
*国家重点研发计划2016YF131200401)、国家自热科基会(51505066)、中央高校基本科研业务经费(ZYGX2015J081)、中国博士后科孕基金(2016T90842)和渐江省博主后科研项目择优(B5H1502002)资助项目。
20160604收到初稿,20170115收到能改霜万方数据
影响着列车转向架甚至整车的运行情况。因此,对滚动轴承的故障诊断研究具有很大必要性及重要意义。
安全域是一种从域的角度描述轴承安全状态的模型,从轴承的运行状态角度考患,在状态特征变量确定的空间内,用于辨识其运行状态是否在正