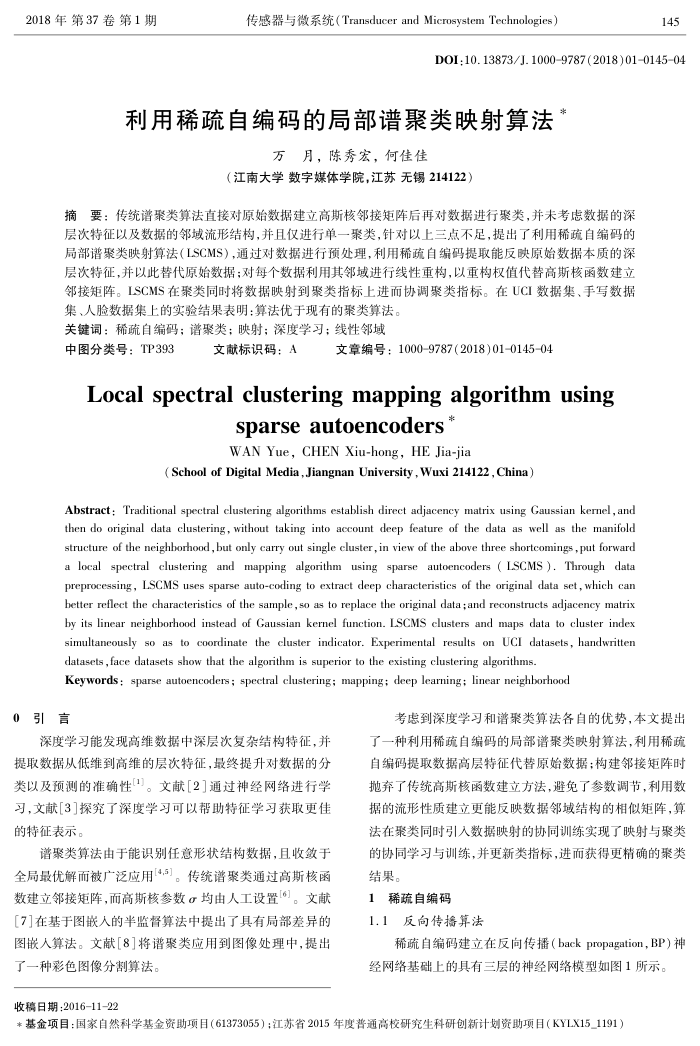
2018年第37卷第1期
传感器与微系统(Transducer andMicrosystemTechnologies)
145
DOI:10.13873/J.10009787(2018)01014504
利用稀疏自编码的局部谱聚类映射算法
万月,陈秀宏,何佳佳
(江南大学数字媒体学院,江苏无锡214122)
摘要:传统谱聚类算法直接对原始数据建立高斯核邻接矩阵后再对数据进行聚类,并未考虑数据的深层次特征以及数据的邻域流形结构,并且仅进行单一聚类,针对以上三点不足,提出了利用稀疏自编码的局部谱聚类映射算法(LSCMS),通过对数据进行预处理,利用稀疏自编码提取能反映原始数据本质的深层次特征,并以此替代原始数据;对母个数据利用其邻域进行线性重构,以重构权值代替高斯核函数建立邻接矩阵。LSCMS在聚类同时将数据映射到聚类指标上进而协调聚类指标。在UCI数据集、手写数据
集、人脸数据集上的实验结果表明:算法优于现有的聚类算法。关键词:稀疏自编码;谱聚类;映射;深度学习;线性邻域
中图分类号:TP393
文献标识码:A
文章编号:1000-9787(2018)01-0145-04
Local spectral clustering mapping algorithm using
sparseautoencoders WAN Yue, CHEN Xiu-hong, HE Jia-jia
( School of Digital Media,Jiangnan University, Wuxi 214122, China)
Abstract: Traditional spectral clustering algorithms establish direct adjacency matrix using Gaussian kernel, and then do original data clustering, without taking into account deep feature of the data as well as the manifold structure of the neighborhood,but only carry out single cluster,in view of the above three shortcomings, put forward a local spectral clustering and mapping algorithm using sparse autoencoders ( LSCMS ). Through data preprocessing, LSCMS uses sparse auto-coding to extract deep characteristics of the original data set, which can better reflect the characteristics of the sample,so as to replace the original data ;and reconstructs adjacency matrix by its linear neighborhood instead of Gaussian kernel function. LSCMS clusters and maps data to cluster index simultaneously so as to coordinate the cluster indicator. Experimental results on UCI datasets, handwritten datasets ,face datasets show that the algorithm is superior to the existing clustering algorithms.
Keywords; sparse autoencoders ; spectral clustering; mapping; deep learning; linear neighborhood
0引言
深度学习能发现高维数据中深层次复杂结构特征,并提取数据从低维到高维的层次特征,最终提升对数据的分类以及预测的准确性""。文献[2]通过神经网络进行学习,文献[3探究了深度学习可以帮助特征学习获取更佳的特征表示。
谱聚类算法由于能识别任意形状结构数据,且收敛于全局最优解而被广泛应用[4,5」。传统谱聚类通过高斯核函数建立邻接矩阵,而高斯核参数α均由人工设置[」。文献[7]在基于图嵌人的半监督算法中提出了具有局部差异的图嵌人算法。文献[8]将谱聚类应用到图像处理中,提出
了一种彩色图像分割算法。收稿日期:2016-11-22
考虑到深度学习和谱聚类算法各自的优势,本文提出了一种利用稀疏自编码的局部谱聚类映射算法,利用稀疏自编码提取数据高层特征代替原始数据;构建邻接矩阵时抛弃了传统高斯核函数建立方法,避免了参数调节,利用数据的流形性质建立更能反映数据邻域结构的相似矩阵,算法在聚类同时引入数据映射的协同训练实现了映射与聚类的协同学习与训练,并更新类指标,进而获得更精确的聚类结果。
1稀疏自编码
1.1反向传播算法
稀疏自编码建立在反向传播(backpropagation,BP)神经网络基础上的具有三层的神经网络模型如图1所示。
*基金项目:国家自然科学基金资助项目(61373055);江苏省2015年度普通高校研究生科研创新计划资助项目(KYLX15_1191)