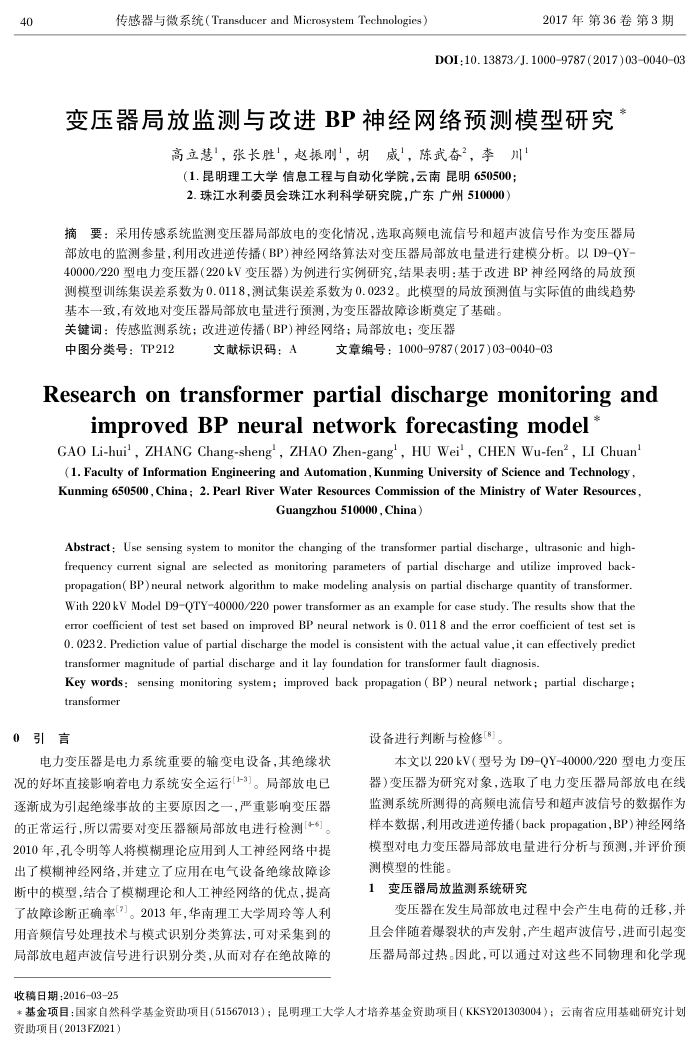
40
传感器与微系统(Transducer and Microsystem Technologies)
2017年第36卷第3期
DOI:10.13873/J.10009787 (2017)03-004003
变压器局放监测与改进BP神经网络预测模型研究
高立慧,张长胜,赵振刚,胡威,陈武奋,李川(1.昆明理工大学信息工程与自动化学院,云南昆明650500; 2.珠江水利委员会珠江水利科学研究院,广东广州510000)
摘要:采用传感系统监测变压器局部放电的变化情况,选取高频电流信号和超声波信号作为变压器局部放电的监测参量,利用改进逆传播(BP)神经网络算法对变压器局部放电量进行建模分析。以D9-QY-40000/220型电力变压器(220kV变压器)为例进行实例研究,结果表明:基手改进BP神经网络的局放预测模型训练集误差系数为0.0118,测试集误差系数为0.0232。此模型的局放预测值与实际值的曲线趋势
基本一致,有效地对变压器局部放电量进行预测,为变压器故障诊断莫定了基础。关键词:传感监测系统;改进逆传播(BP)神经网络;局部放电;变压器
中图分类号:TP212
文献标识码:A
文章编号:1000-9787(2017)03-0040-03
Research on transformer partial discharge monitoring and
improved BP neural network forecasting model*
GAO Li-hui', ZHANG Chang-sheng', ZHAO Zhen-gang', HU Wei', CHEN Wu-fen’, LI Chuan(1.Faculty of Information Engineering and Automation, Kunming University of Science and Technology, Kunming 650500, China; 2. Pearl River Water Resources Commission of the Ministry of Water Resources
Guangzhou510000,China)
Abstract: Use sensing system to monitor the changing of the transfomer partial discharge, ultrasonic and high-frequency current signal are selected as monitoring parameters of partial discharge and utilize improved back-propagation( BP) neural network algorithm to make modeling analysis on partial discharge quantity of transformer. With 220 kV Model D9QTY40000/220 power transformer as an example for case study. The results show that the error coefficient of test set based on improved BP neural network is 0. 011 8 and the error coefficient of test set is 0. 023 2. Prediction value of partial discharge the model is consistent with the actual value ,it can effectively predict transformer magnitude of partial discharge and it lay foundation for transformer fault diagnosis.
Key words: sensing monitoring system; improved back propagation ( BP) neural network; partial discharge; transfomer
0引言
电力变压器是电力系统重要的输变电设备,其绝缘状况的好坏直接影响着电力系统安全运行[1-3]。局部放电已逐渐成为引起绝缘事故的主要原因之一,严重影响变压器的正常运行,所以需要对变压器额局部放电进行检测[46]。 2010年,孔令明等人将模糊理论应用到人工神经网络中提出了模糊神经网络,并建立了应用在电气设备绝缘故障诊断申的模型,结合广模糊理论和人工神经网络的优点,提高了故障诊断正确率7。2013年,华南理工大学周玲等人利用音频信号处理技术与模式识别分类算法,可对采集到的局部放电超声波信号进行识别分类,从而对存在绝故障的
收稿日期:2016-03-25
设备进行判断与检修(8)。
本文以220kV(型号为D9-QY-40000/220型电力变压器)变压器为研究对象,选取了电力变压器局部放电在线监测系统所测得的高频电流信号和超声波信号的数据作为样本数据,利用改进逆传播(backpropagation,BP)神经网络模型对电力变压器局部放电量进行分析与预测,并评价预测模型的性能。
变压器局放监测系统研究 1
变压器在发生局部放电过程中会产生电荷的迁移,并且会伴随者爆裂状的声发射,产生超声波信号,进而弓引起变压器局部过热。因此,可以通过对这些不同物理和化学现
*基金项目:国家自然科学基金资助项目(51567013);昆明理工大学人才培养基金资助项目(KKSY201303004);云南省应用基础研究计划资助项目(2013FZ021)